Least Squares: Is there a way to improve the performance of this code? packages used: sm.OLS and pd
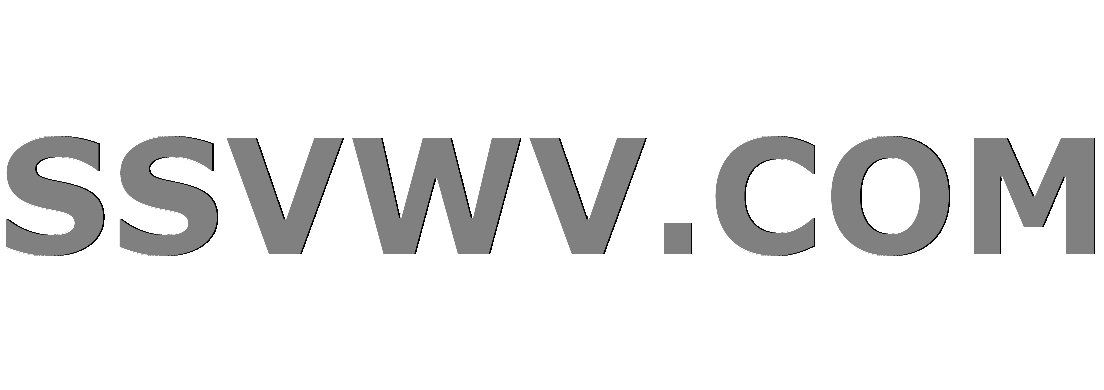
Multi tool use
Following function calculates the slope and intercept from a pandas data frame. For big data the calculations takes too long.
import pandas as pd
import statsmodels.api as sm
df = pd.DataFrame({'signal': [270400, 270320, 270278.2609, 270195.8333, 270207.1429, 270081.8182, 269891.3043, 269991.6667, 270153.3333, 270285, 270357.1429, 270485.7143, 270526.3158, 270422.7273, 270310.7143, 270486.3636, 270593.9394, 270655.5556, 270669.2308, 270800, 270911.1111, 271083.3333, 271180, 271084.6154, 270800, 270966.6667, 271100, 271030.7692, 271053.8462, 271123.8095, 271050, 271165.5172, 271384, 271486.6667, 271554.5455, 271600, 271629.1667, 271526.6667, 271612.5, 271663.6364, 271553.5714, 271384, 271400, 271676.9231, 271666.6667, 271624, 271540.7407, 271503.125, 271600, 271620.8333, 271426.087, 271492.8571, 271482.1429, 271426.3158, 271207.6923, 271146.6667, 271057.8947, 271000, 271005.5556, 271044, 270930.4348, 270707.6923, 270833.3333, 270950, 270912.5, 270850, 270711.1111, 270925.9259, 271270, 271220, 271050, 271069.2308, 271037.5, 270954.5455, 270720, 270631.25, 270450, 270387.5, 270196, 270271.4286, 269920, 269760, 269562.5, 269500, 269867.8571, 269792.8571, 269854.1667, 269666.6667, 269417.3913, 269183.3333, 268966.6667, 268859.0909, 268707.6923, 268634.4828, 268516.6667, 268500, 268753.3333, 269175, 269309.5238, 269440.9091, 269394.4444, 269147.3684, 269133.3333, 269083.3333, 269126.087, 269145, 269164.7059, 269175, 269247.3684, 269540.9091, 270039.2857, 270150, 270283.3333, 270480.9524, 270692.8571, 270350, 270414.2857, 270277.7778, 270359.2593, 270148.1481, 269905, 269800, 269731.8182, 270186.6667, 270271.4286, 269983.3333, 269808, 269900, 269652.381, 269434.6154, 269659.2593, 269770.9677, 269831.0345, 269960, 270000, 270021.7391, 269868.4211, 269421.0526, 269106.25, 268813.3333, 268685.7143, 269421.4286, 269712.5, 269546.1538, 269332.2581, 269103.3333, 269289.2857, 269493.5484, 269650, 269710, 269444.4444, 269727.2727, 269833.3333, 269844.4444, 269985.7143, 270445.4545, 270320, 270092.5926, 269978.9474, 269866.6667, 269666.6667, 269468.75, 269582.3529, 269300, 269336.3636, 269309.5238, 269233.3333, 269208, 269100] })
def OLS_slope_intercept(df,select_colname='open'):
model = sm.OLS(df[select_colname], sm.add_constant(df.index)).fit()
intercept = model.params[0]
slope = model.params[1]
return slope, intercept
import time
start = time.time()
slope, intercept = OLS_slope_intercept(df,'signal')
end= time.time()
print('running time of code:' +str(end-start))
python-3.x pandas performance statsmodels least-squares
add a comment |
Following function calculates the slope and intercept from a pandas data frame. For big data the calculations takes too long.
import pandas as pd
import statsmodels.api as sm
df = pd.DataFrame({'signal': [270400, 270320, 270278.2609, 270195.8333, 270207.1429, 270081.8182, 269891.3043, 269991.6667, 270153.3333, 270285, 270357.1429, 270485.7143, 270526.3158, 270422.7273, 270310.7143, 270486.3636, 270593.9394, 270655.5556, 270669.2308, 270800, 270911.1111, 271083.3333, 271180, 271084.6154, 270800, 270966.6667, 271100, 271030.7692, 271053.8462, 271123.8095, 271050, 271165.5172, 271384, 271486.6667, 271554.5455, 271600, 271629.1667, 271526.6667, 271612.5, 271663.6364, 271553.5714, 271384, 271400, 271676.9231, 271666.6667, 271624, 271540.7407, 271503.125, 271600, 271620.8333, 271426.087, 271492.8571, 271482.1429, 271426.3158, 271207.6923, 271146.6667, 271057.8947, 271000, 271005.5556, 271044, 270930.4348, 270707.6923, 270833.3333, 270950, 270912.5, 270850, 270711.1111, 270925.9259, 271270, 271220, 271050, 271069.2308, 271037.5, 270954.5455, 270720, 270631.25, 270450, 270387.5, 270196, 270271.4286, 269920, 269760, 269562.5, 269500, 269867.8571, 269792.8571, 269854.1667, 269666.6667, 269417.3913, 269183.3333, 268966.6667, 268859.0909, 268707.6923, 268634.4828, 268516.6667, 268500, 268753.3333, 269175, 269309.5238, 269440.9091, 269394.4444, 269147.3684, 269133.3333, 269083.3333, 269126.087, 269145, 269164.7059, 269175, 269247.3684, 269540.9091, 270039.2857, 270150, 270283.3333, 270480.9524, 270692.8571, 270350, 270414.2857, 270277.7778, 270359.2593, 270148.1481, 269905, 269800, 269731.8182, 270186.6667, 270271.4286, 269983.3333, 269808, 269900, 269652.381, 269434.6154, 269659.2593, 269770.9677, 269831.0345, 269960, 270000, 270021.7391, 269868.4211, 269421.0526, 269106.25, 268813.3333, 268685.7143, 269421.4286, 269712.5, 269546.1538, 269332.2581, 269103.3333, 269289.2857, 269493.5484, 269650, 269710, 269444.4444, 269727.2727, 269833.3333, 269844.4444, 269985.7143, 270445.4545, 270320, 270092.5926, 269978.9474, 269866.6667, 269666.6667, 269468.75, 269582.3529, 269300, 269336.3636, 269309.5238, 269233.3333, 269208, 269100] })
def OLS_slope_intercept(df,select_colname='open'):
model = sm.OLS(df[select_colname], sm.add_constant(df.index)).fit()
intercept = model.params[0]
slope = model.params[1]
return slope, intercept
import time
start = time.time()
slope, intercept = OLS_slope_intercept(df,'signal')
end= time.time()
print('running time of code:' +str(end-start))
python-3.x pandas performance statsmodels least-squares
Both answers improve the performance significantly.
– mqx
Nov 11 '18 at 2:14
add a comment |
Following function calculates the slope and intercept from a pandas data frame. For big data the calculations takes too long.
import pandas as pd
import statsmodels.api as sm
df = pd.DataFrame({'signal': [270400, 270320, 270278.2609, 270195.8333, 270207.1429, 270081.8182, 269891.3043, 269991.6667, 270153.3333, 270285, 270357.1429, 270485.7143, 270526.3158, 270422.7273, 270310.7143, 270486.3636, 270593.9394, 270655.5556, 270669.2308, 270800, 270911.1111, 271083.3333, 271180, 271084.6154, 270800, 270966.6667, 271100, 271030.7692, 271053.8462, 271123.8095, 271050, 271165.5172, 271384, 271486.6667, 271554.5455, 271600, 271629.1667, 271526.6667, 271612.5, 271663.6364, 271553.5714, 271384, 271400, 271676.9231, 271666.6667, 271624, 271540.7407, 271503.125, 271600, 271620.8333, 271426.087, 271492.8571, 271482.1429, 271426.3158, 271207.6923, 271146.6667, 271057.8947, 271000, 271005.5556, 271044, 270930.4348, 270707.6923, 270833.3333, 270950, 270912.5, 270850, 270711.1111, 270925.9259, 271270, 271220, 271050, 271069.2308, 271037.5, 270954.5455, 270720, 270631.25, 270450, 270387.5, 270196, 270271.4286, 269920, 269760, 269562.5, 269500, 269867.8571, 269792.8571, 269854.1667, 269666.6667, 269417.3913, 269183.3333, 268966.6667, 268859.0909, 268707.6923, 268634.4828, 268516.6667, 268500, 268753.3333, 269175, 269309.5238, 269440.9091, 269394.4444, 269147.3684, 269133.3333, 269083.3333, 269126.087, 269145, 269164.7059, 269175, 269247.3684, 269540.9091, 270039.2857, 270150, 270283.3333, 270480.9524, 270692.8571, 270350, 270414.2857, 270277.7778, 270359.2593, 270148.1481, 269905, 269800, 269731.8182, 270186.6667, 270271.4286, 269983.3333, 269808, 269900, 269652.381, 269434.6154, 269659.2593, 269770.9677, 269831.0345, 269960, 270000, 270021.7391, 269868.4211, 269421.0526, 269106.25, 268813.3333, 268685.7143, 269421.4286, 269712.5, 269546.1538, 269332.2581, 269103.3333, 269289.2857, 269493.5484, 269650, 269710, 269444.4444, 269727.2727, 269833.3333, 269844.4444, 269985.7143, 270445.4545, 270320, 270092.5926, 269978.9474, 269866.6667, 269666.6667, 269468.75, 269582.3529, 269300, 269336.3636, 269309.5238, 269233.3333, 269208, 269100] })
def OLS_slope_intercept(df,select_colname='open'):
model = sm.OLS(df[select_colname], sm.add_constant(df.index)).fit()
intercept = model.params[0]
slope = model.params[1]
return slope, intercept
import time
start = time.time()
slope, intercept = OLS_slope_intercept(df,'signal')
end= time.time()
print('running time of code:' +str(end-start))
python-3.x pandas performance statsmodels least-squares
Following function calculates the slope and intercept from a pandas data frame. For big data the calculations takes too long.
import pandas as pd
import statsmodels.api as sm
df = pd.DataFrame({'signal': [270400, 270320, 270278.2609, 270195.8333, 270207.1429, 270081.8182, 269891.3043, 269991.6667, 270153.3333, 270285, 270357.1429, 270485.7143, 270526.3158, 270422.7273, 270310.7143, 270486.3636, 270593.9394, 270655.5556, 270669.2308, 270800, 270911.1111, 271083.3333, 271180, 271084.6154, 270800, 270966.6667, 271100, 271030.7692, 271053.8462, 271123.8095, 271050, 271165.5172, 271384, 271486.6667, 271554.5455, 271600, 271629.1667, 271526.6667, 271612.5, 271663.6364, 271553.5714, 271384, 271400, 271676.9231, 271666.6667, 271624, 271540.7407, 271503.125, 271600, 271620.8333, 271426.087, 271492.8571, 271482.1429, 271426.3158, 271207.6923, 271146.6667, 271057.8947, 271000, 271005.5556, 271044, 270930.4348, 270707.6923, 270833.3333, 270950, 270912.5, 270850, 270711.1111, 270925.9259, 271270, 271220, 271050, 271069.2308, 271037.5, 270954.5455, 270720, 270631.25, 270450, 270387.5, 270196, 270271.4286, 269920, 269760, 269562.5, 269500, 269867.8571, 269792.8571, 269854.1667, 269666.6667, 269417.3913, 269183.3333, 268966.6667, 268859.0909, 268707.6923, 268634.4828, 268516.6667, 268500, 268753.3333, 269175, 269309.5238, 269440.9091, 269394.4444, 269147.3684, 269133.3333, 269083.3333, 269126.087, 269145, 269164.7059, 269175, 269247.3684, 269540.9091, 270039.2857, 270150, 270283.3333, 270480.9524, 270692.8571, 270350, 270414.2857, 270277.7778, 270359.2593, 270148.1481, 269905, 269800, 269731.8182, 270186.6667, 270271.4286, 269983.3333, 269808, 269900, 269652.381, 269434.6154, 269659.2593, 269770.9677, 269831.0345, 269960, 270000, 270021.7391, 269868.4211, 269421.0526, 269106.25, 268813.3333, 268685.7143, 269421.4286, 269712.5, 269546.1538, 269332.2581, 269103.3333, 269289.2857, 269493.5484, 269650, 269710, 269444.4444, 269727.2727, 269833.3333, 269844.4444, 269985.7143, 270445.4545, 270320, 270092.5926, 269978.9474, 269866.6667, 269666.6667, 269468.75, 269582.3529, 269300, 269336.3636, 269309.5238, 269233.3333, 269208, 269100] })
def OLS_slope_intercept(df,select_colname='open'):
model = sm.OLS(df[select_colname], sm.add_constant(df.index)).fit()
intercept = model.params[0]
slope = model.params[1]
return slope, intercept
import time
start = time.time()
slope, intercept = OLS_slope_intercept(df,'signal')
end= time.time()
print('running time of code:' +str(end-start))
python-3.x pandas performance statsmodels least-squares
python-3.x pandas performance statsmodels least-squares
edited Nov 10 '18 at 17:30
mqx
asked Nov 10 '18 at 15:10


mqxmqx
64
64
Both answers improve the performance significantly.
– mqx
Nov 11 '18 at 2:14
add a comment |
Both answers improve the performance significantly.
– mqx
Nov 11 '18 at 2:14
Both answers improve the performance significantly.
– mqx
Nov 11 '18 at 2:14
Both answers improve the performance significantly.
– mqx
Nov 11 '18 at 2:14
add a comment |
2 Answers
2
active
oldest
votes
You can try scipy.stats.linregress
which is faster than statsmodels
:
import pandas as pd
from scipy.stats import linregress
import statsmodels.api as sm
import numpy as np
def OLS_slope_intercept(df,select_colname='open'):
model = sm.OLS(df[select_colname], sm.add_constant(df.index)).fit()
intercept = model.params[0]
slope = model.params[1]
return slope, intercept
import time
for i in [10,100,1000,10000,100000]:
df1 = pd.concat([df]*i)
start = time.time()
slope, intercept = OLS_slope_intercept(df1,'signal')
end= time.time()
print('running time of for STATSMODEL Library: ' +str(end-start))
start = time.time()
slope, intercept, r_value, p_value, std_err = linregress(df1.index,df1.signal)
end= time.time()
print('running time of for SCIPY Library code: ' +str(end-start))
start = time.time()
coeffs = np.polyfit(df1.index, df1.signal, 1) # 1=linear
end= time.time()
print('running time of for Numpy Library code: ' +str(end-start),'n')
running time of for STATSMODEL Library: 0.018347978591918945
running time of for SCIPY Library code: 0.0010001659393310547
running time of for Numpy Library code: 0.0009999275207519531
running time of for STATSMODEL Library: 0.005003690719604492
running time of for SCIPY Library code: 0.0010006427764892578
running time of for Numpy Library code: 0.0010006427764892578
running time of for STATSMODEL Library: 0.033023834228515625
running time of for SCIPY Library code: 0.0010905265808105469
running time of for Numpy Library code: 0.0
running time of for STATSMODEL Library: 0.2552676200866699
running time of for SCIPY Library code: 0.050981998443603516
running time of for Numpy Library code: 0.11161375045776367
running time of for STATSMODEL Library: 2.6753437519073486
running time of for SCIPY Library code: 0.3832666873931885
running time of for Numpy Library code: 1.2618811130523682
My conclusion: On average np.polyfit is 5% faster than linregress from scipy.stats.
– mqx
Nov 13 '18 at 0:05
@mqx check the updatelinegress
is faster thannp.ployfit
.
– Sandeep Kadapa
Nov 13 '18 at 3:54
add a comment |
You can just use numpy.polyfit()
import numpy as np
import time
start = time.time()
coeffs = np.polyfit(df.index, df.signal, 1) # 1=linear
end= time.time()
slope, intercept = coeffs
print(f'Run Time: {end-start}nSlope: {slope}nIntercept: {intercept}')
Run Time: 0.000385284423828125
Slope: -10.83867485480565
Intercept: 271144.8357256735
When I run your example running time of code:0.002992868423461914
add a comment |
Your Answer
StackExchange.ifUsing("editor", function () {
StackExchange.using("externalEditor", function () {
StackExchange.using("snippets", function () {
StackExchange.snippets.init();
});
});
}, "code-snippets");
StackExchange.ready(function() {
var channelOptions = {
tags: "".split(" "),
id: "1"
};
initTagRenderer("".split(" "), "".split(" "), channelOptions);
StackExchange.using("externalEditor", function() {
// Have to fire editor after snippets, if snippets enabled
if (StackExchange.settings.snippets.snippetsEnabled) {
StackExchange.using("snippets", function() {
createEditor();
});
}
else {
createEditor();
}
});
function createEditor() {
StackExchange.prepareEditor({
heartbeatType: 'answer',
autoActivateHeartbeat: false,
convertImagesToLinks: true,
noModals: true,
showLowRepImageUploadWarning: true,
reputationToPostImages: 10,
bindNavPrevention: true,
postfix: "",
imageUploader: {
brandingHtml: "Powered by u003ca class="icon-imgur-white" href="https://imgur.com/"u003eu003c/au003e",
contentPolicyHtml: "User contributions licensed under u003ca href="https://creativecommons.org/licenses/by-sa/3.0/"u003ecc by-sa 3.0 with attribution requiredu003c/au003e u003ca href="https://stackoverflow.com/legal/content-policy"u003e(content policy)u003c/au003e",
allowUrls: true
},
onDemand: true,
discardSelector: ".discard-answer"
,immediatelyShowMarkdownHelp:true
});
}
});
Sign up or log in
StackExchange.ready(function () {
StackExchange.helpers.onClickDraftSave('#login-link');
});
Sign up using Google
Sign up using Facebook
Sign up using Email and Password
Post as a guest
Required, but never shown
StackExchange.ready(
function () {
StackExchange.openid.initPostLogin('.new-post-login', 'https%3a%2f%2fstackoverflow.com%2fquestions%2f53240267%2fleast-squares-is-there-a-way-to-improve-the-performance-of-this-code-packages%23new-answer', 'question_page');
}
);
Post as a guest
Required, but never shown
2 Answers
2
active
oldest
votes
2 Answers
2
active
oldest
votes
active
oldest
votes
active
oldest
votes
You can try scipy.stats.linregress
which is faster than statsmodels
:
import pandas as pd
from scipy.stats import linregress
import statsmodels.api as sm
import numpy as np
def OLS_slope_intercept(df,select_colname='open'):
model = sm.OLS(df[select_colname], sm.add_constant(df.index)).fit()
intercept = model.params[0]
slope = model.params[1]
return slope, intercept
import time
for i in [10,100,1000,10000,100000]:
df1 = pd.concat([df]*i)
start = time.time()
slope, intercept = OLS_slope_intercept(df1,'signal')
end= time.time()
print('running time of for STATSMODEL Library: ' +str(end-start))
start = time.time()
slope, intercept, r_value, p_value, std_err = linregress(df1.index,df1.signal)
end= time.time()
print('running time of for SCIPY Library code: ' +str(end-start))
start = time.time()
coeffs = np.polyfit(df1.index, df1.signal, 1) # 1=linear
end= time.time()
print('running time of for Numpy Library code: ' +str(end-start),'n')
running time of for STATSMODEL Library: 0.018347978591918945
running time of for SCIPY Library code: 0.0010001659393310547
running time of for Numpy Library code: 0.0009999275207519531
running time of for STATSMODEL Library: 0.005003690719604492
running time of for SCIPY Library code: 0.0010006427764892578
running time of for Numpy Library code: 0.0010006427764892578
running time of for STATSMODEL Library: 0.033023834228515625
running time of for SCIPY Library code: 0.0010905265808105469
running time of for Numpy Library code: 0.0
running time of for STATSMODEL Library: 0.2552676200866699
running time of for SCIPY Library code: 0.050981998443603516
running time of for Numpy Library code: 0.11161375045776367
running time of for STATSMODEL Library: 2.6753437519073486
running time of for SCIPY Library code: 0.3832666873931885
running time of for Numpy Library code: 1.2618811130523682
My conclusion: On average np.polyfit is 5% faster than linregress from scipy.stats.
– mqx
Nov 13 '18 at 0:05
@mqx check the updatelinegress
is faster thannp.ployfit
.
– Sandeep Kadapa
Nov 13 '18 at 3:54
add a comment |
You can try scipy.stats.linregress
which is faster than statsmodels
:
import pandas as pd
from scipy.stats import linregress
import statsmodels.api as sm
import numpy as np
def OLS_slope_intercept(df,select_colname='open'):
model = sm.OLS(df[select_colname], sm.add_constant(df.index)).fit()
intercept = model.params[0]
slope = model.params[1]
return slope, intercept
import time
for i in [10,100,1000,10000,100000]:
df1 = pd.concat([df]*i)
start = time.time()
slope, intercept = OLS_slope_intercept(df1,'signal')
end= time.time()
print('running time of for STATSMODEL Library: ' +str(end-start))
start = time.time()
slope, intercept, r_value, p_value, std_err = linregress(df1.index,df1.signal)
end= time.time()
print('running time of for SCIPY Library code: ' +str(end-start))
start = time.time()
coeffs = np.polyfit(df1.index, df1.signal, 1) # 1=linear
end= time.time()
print('running time of for Numpy Library code: ' +str(end-start),'n')
running time of for STATSMODEL Library: 0.018347978591918945
running time of for SCIPY Library code: 0.0010001659393310547
running time of for Numpy Library code: 0.0009999275207519531
running time of for STATSMODEL Library: 0.005003690719604492
running time of for SCIPY Library code: 0.0010006427764892578
running time of for Numpy Library code: 0.0010006427764892578
running time of for STATSMODEL Library: 0.033023834228515625
running time of for SCIPY Library code: 0.0010905265808105469
running time of for Numpy Library code: 0.0
running time of for STATSMODEL Library: 0.2552676200866699
running time of for SCIPY Library code: 0.050981998443603516
running time of for Numpy Library code: 0.11161375045776367
running time of for STATSMODEL Library: 2.6753437519073486
running time of for SCIPY Library code: 0.3832666873931885
running time of for Numpy Library code: 1.2618811130523682
My conclusion: On average np.polyfit is 5% faster than linregress from scipy.stats.
– mqx
Nov 13 '18 at 0:05
@mqx check the updatelinegress
is faster thannp.ployfit
.
– Sandeep Kadapa
Nov 13 '18 at 3:54
add a comment |
You can try scipy.stats.linregress
which is faster than statsmodels
:
import pandas as pd
from scipy.stats import linregress
import statsmodels.api as sm
import numpy as np
def OLS_slope_intercept(df,select_colname='open'):
model = sm.OLS(df[select_colname], sm.add_constant(df.index)).fit()
intercept = model.params[0]
slope = model.params[1]
return slope, intercept
import time
for i in [10,100,1000,10000,100000]:
df1 = pd.concat([df]*i)
start = time.time()
slope, intercept = OLS_slope_intercept(df1,'signal')
end= time.time()
print('running time of for STATSMODEL Library: ' +str(end-start))
start = time.time()
slope, intercept, r_value, p_value, std_err = linregress(df1.index,df1.signal)
end= time.time()
print('running time of for SCIPY Library code: ' +str(end-start))
start = time.time()
coeffs = np.polyfit(df1.index, df1.signal, 1) # 1=linear
end= time.time()
print('running time of for Numpy Library code: ' +str(end-start),'n')
running time of for STATSMODEL Library: 0.018347978591918945
running time of for SCIPY Library code: 0.0010001659393310547
running time of for Numpy Library code: 0.0009999275207519531
running time of for STATSMODEL Library: 0.005003690719604492
running time of for SCIPY Library code: 0.0010006427764892578
running time of for Numpy Library code: 0.0010006427764892578
running time of for STATSMODEL Library: 0.033023834228515625
running time of for SCIPY Library code: 0.0010905265808105469
running time of for Numpy Library code: 0.0
running time of for STATSMODEL Library: 0.2552676200866699
running time of for SCIPY Library code: 0.050981998443603516
running time of for Numpy Library code: 0.11161375045776367
running time of for STATSMODEL Library: 2.6753437519073486
running time of for SCIPY Library code: 0.3832666873931885
running time of for Numpy Library code: 1.2618811130523682
You can try scipy.stats.linregress
which is faster than statsmodels
:
import pandas as pd
from scipy.stats import linregress
import statsmodels.api as sm
import numpy as np
def OLS_slope_intercept(df,select_colname='open'):
model = sm.OLS(df[select_colname], sm.add_constant(df.index)).fit()
intercept = model.params[0]
slope = model.params[1]
return slope, intercept
import time
for i in [10,100,1000,10000,100000]:
df1 = pd.concat([df]*i)
start = time.time()
slope, intercept = OLS_slope_intercept(df1,'signal')
end= time.time()
print('running time of for STATSMODEL Library: ' +str(end-start))
start = time.time()
slope, intercept, r_value, p_value, std_err = linregress(df1.index,df1.signal)
end= time.time()
print('running time of for SCIPY Library code: ' +str(end-start))
start = time.time()
coeffs = np.polyfit(df1.index, df1.signal, 1) # 1=linear
end= time.time()
print('running time of for Numpy Library code: ' +str(end-start),'n')
running time of for STATSMODEL Library: 0.018347978591918945
running time of for SCIPY Library code: 0.0010001659393310547
running time of for Numpy Library code: 0.0009999275207519531
running time of for STATSMODEL Library: 0.005003690719604492
running time of for SCIPY Library code: 0.0010006427764892578
running time of for Numpy Library code: 0.0010006427764892578
running time of for STATSMODEL Library: 0.033023834228515625
running time of for SCIPY Library code: 0.0010905265808105469
running time of for Numpy Library code: 0.0
running time of for STATSMODEL Library: 0.2552676200866699
running time of for SCIPY Library code: 0.050981998443603516
running time of for Numpy Library code: 0.11161375045776367
running time of for STATSMODEL Library: 2.6753437519073486
running time of for SCIPY Library code: 0.3832666873931885
running time of for Numpy Library code: 1.2618811130523682
edited Nov 13 '18 at 15:40
answered Nov 10 '18 at 16:01


Sandeep KadapaSandeep Kadapa
6,302429
6,302429
My conclusion: On average np.polyfit is 5% faster than linregress from scipy.stats.
– mqx
Nov 13 '18 at 0:05
@mqx check the updatelinegress
is faster thannp.ployfit
.
– Sandeep Kadapa
Nov 13 '18 at 3:54
add a comment |
My conclusion: On average np.polyfit is 5% faster than linregress from scipy.stats.
– mqx
Nov 13 '18 at 0:05
@mqx check the updatelinegress
is faster thannp.ployfit
.
– Sandeep Kadapa
Nov 13 '18 at 3:54
My conclusion: On average np.polyfit is 5% faster than linregress from scipy.stats.
– mqx
Nov 13 '18 at 0:05
My conclusion: On average np.polyfit is 5% faster than linregress from scipy.stats.
– mqx
Nov 13 '18 at 0:05
@mqx check the update
linegress
is faster than np.ployfit
.– Sandeep Kadapa
Nov 13 '18 at 3:54
@mqx check the update
linegress
is faster than np.ployfit
.– Sandeep Kadapa
Nov 13 '18 at 3:54
add a comment |
You can just use numpy.polyfit()
import numpy as np
import time
start = time.time()
coeffs = np.polyfit(df.index, df.signal, 1) # 1=linear
end= time.time()
slope, intercept = coeffs
print(f'Run Time: {end-start}nSlope: {slope}nIntercept: {intercept}')
Run Time: 0.000385284423828125
Slope: -10.83867485480565
Intercept: 271144.8357256735
When I run your example running time of code:0.002992868423461914
add a comment |
You can just use numpy.polyfit()
import numpy as np
import time
start = time.time()
coeffs = np.polyfit(df.index, df.signal, 1) # 1=linear
end= time.time()
slope, intercept = coeffs
print(f'Run Time: {end-start}nSlope: {slope}nIntercept: {intercept}')
Run Time: 0.000385284423828125
Slope: -10.83867485480565
Intercept: 271144.8357256735
When I run your example running time of code:0.002992868423461914
add a comment |
You can just use numpy.polyfit()
import numpy as np
import time
start = time.time()
coeffs = np.polyfit(df.index, df.signal, 1) # 1=linear
end= time.time()
slope, intercept = coeffs
print(f'Run Time: {end-start}nSlope: {slope}nIntercept: {intercept}')
Run Time: 0.000385284423828125
Slope: -10.83867485480565
Intercept: 271144.8357256735
When I run your example running time of code:0.002992868423461914
You can just use numpy.polyfit()
import numpy as np
import time
start = time.time()
coeffs = np.polyfit(df.index, df.signal, 1) # 1=linear
end= time.time()
slope, intercept = coeffs
print(f'Run Time: {end-start}nSlope: {slope}nIntercept: {intercept}')
Run Time: 0.000385284423828125
Slope: -10.83867485480565
Intercept: 271144.8357256735
When I run your example running time of code:0.002992868423461914
answered Nov 10 '18 at 15:45
ChrisChris
2,0411318
2,0411318
add a comment |
add a comment |
Thanks for contributing an answer to Stack Overflow!
- Please be sure to answer the question. Provide details and share your research!
But avoid …
- Asking for help, clarification, or responding to other answers.
- Making statements based on opinion; back them up with references or personal experience.
To learn more, see our tips on writing great answers.
Sign up or log in
StackExchange.ready(function () {
StackExchange.helpers.onClickDraftSave('#login-link');
});
Sign up using Google
Sign up using Facebook
Sign up using Email and Password
Post as a guest
Required, but never shown
StackExchange.ready(
function () {
StackExchange.openid.initPostLogin('.new-post-login', 'https%3a%2f%2fstackoverflow.com%2fquestions%2f53240267%2fleast-squares-is-there-a-way-to-improve-the-performance-of-this-code-packages%23new-answer', 'question_page');
}
);
Post as a guest
Required, but never shown
Sign up or log in
StackExchange.ready(function () {
StackExchange.helpers.onClickDraftSave('#login-link');
});
Sign up using Google
Sign up using Facebook
Sign up using Email and Password
Post as a guest
Required, but never shown
Sign up or log in
StackExchange.ready(function () {
StackExchange.helpers.onClickDraftSave('#login-link');
});
Sign up using Google
Sign up using Facebook
Sign up using Email and Password
Post as a guest
Required, but never shown
Sign up or log in
StackExchange.ready(function () {
StackExchange.helpers.onClickDraftSave('#login-link');
});
Sign up using Google
Sign up using Facebook
Sign up using Email and Password
Sign up using Google
Sign up using Facebook
Sign up using Email and Password
Post as a guest
Required, but never shown
Required, but never shown
Required, but never shown
Required, but never shown
Required, but never shown
Required, but never shown
Required, but never shown
Required, but never shown
Required, but never shown
xSuQhRow
Both answers improve the performance significantly.
– mqx
Nov 11 '18 at 2:14