How to Update parameters every certain steps?
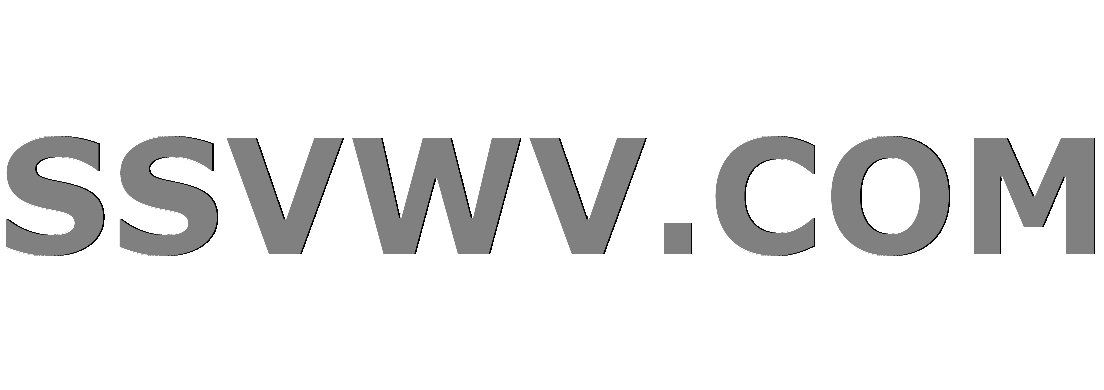
Multi tool use
I'm using a tensorflow based keras to train a object detection network.
Due to small GPU memory size, have to update params once per some steps to "expand" batch size.
But my implementation can't successfully converge in Mask Rcnn training or perform badly comparing normal optimizers in simple cifar10 classification:
class custom_SGD(Optimizer):
def get_updates(self, loss, params):
"""Main params updates operation
"""
shapes = [K.int_shape(p) for p in params]
if self.nesterov:
sum_grads = [K.zeros(shape) for shape in shapes]
moments = [K.zeros(shape) for shape in shapes]
sum_moments = [K.zeros(shape) for shape in shapes]
# Current gradients
grads = self.get_gradients(loss, params)
self.updates = [K.update_add(self.iterations, 1)]
self.weights = [self.iterations] + moments
cond1 = K.equal(self.iterations % self.steps_per_update, 0)
# Learning rate decay
lr = self.lr
if self.initial_decay > 0:
lr = lr * (1. / (1. + self.decay * K.cast(self.iterations,
K.dtype(self.decay))))
if not self.nesterov:
for p, g, m,sm in zip(params, grads,moments, sum_moments):
v = self.momentum*m - lr*g
# updates sum_moments, moments
self.updates.append(K.update(sm, sm+v))
self.updates.append(K.switch(cond1,K.update(m,sm/float(self.steps_per_update)),m))
new_p = p + m
# Apply constraint
if getattr(p, 'constraint', None) is not None:
new_p = p.constraint(new_p)
self.updates.append(K.switch(cond1,K.update(p,new_p),p))
# Clear up container
self.updates.append(K.switch(cond1,K.update(sm,K.zeros_like(sm)),sm))
return self.updates
Can anybody tell me why.
Thanks in advance.
tensorflow keras deep-learning
add a comment |
I'm using a tensorflow based keras to train a object detection network.
Due to small GPU memory size, have to update params once per some steps to "expand" batch size.
But my implementation can't successfully converge in Mask Rcnn training or perform badly comparing normal optimizers in simple cifar10 classification:
class custom_SGD(Optimizer):
def get_updates(self, loss, params):
"""Main params updates operation
"""
shapes = [K.int_shape(p) for p in params]
if self.nesterov:
sum_grads = [K.zeros(shape) for shape in shapes]
moments = [K.zeros(shape) for shape in shapes]
sum_moments = [K.zeros(shape) for shape in shapes]
# Current gradients
grads = self.get_gradients(loss, params)
self.updates = [K.update_add(self.iterations, 1)]
self.weights = [self.iterations] + moments
cond1 = K.equal(self.iterations % self.steps_per_update, 0)
# Learning rate decay
lr = self.lr
if self.initial_decay > 0:
lr = lr * (1. / (1. + self.decay * K.cast(self.iterations,
K.dtype(self.decay))))
if not self.nesterov:
for p, g, m,sm in zip(params, grads,moments, sum_moments):
v = self.momentum*m - lr*g
# updates sum_moments, moments
self.updates.append(K.update(sm, sm+v))
self.updates.append(K.switch(cond1,K.update(m,sm/float(self.steps_per_update)),m))
new_p = p + m
# Apply constraint
if getattr(p, 'constraint', None) is not None:
new_p = p.constraint(new_p)
self.updates.append(K.switch(cond1,K.update(p,new_p),p))
# Clear up container
self.updates.append(K.switch(cond1,K.update(sm,K.zeros_like(sm)),sm))
return self.updates
Can anybody tell me why.
Thanks in advance.
tensorflow keras deep-learning
add a comment |
I'm using a tensorflow based keras to train a object detection network.
Due to small GPU memory size, have to update params once per some steps to "expand" batch size.
But my implementation can't successfully converge in Mask Rcnn training or perform badly comparing normal optimizers in simple cifar10 classification:
class custom_SGD(Optimizer):
def get_updates(self, loss, params):
"""Main params updates operation
"""
shapes = [K.int_shape(p) for p in params]
if self.nesterov:
sum_grads = [K.zeros(shape) for shape in shapes]
moments = [K.zeros(shape) for shape in shapes]
sum_moments = [K.zeros(shape) for shape in shapes]
# Current gradients
grads = self.get_gradients(loss, params)
self.updates = [K.update_add(self.iterations, 1)]
self.weights = [self.iterations] + moments
cond1 = K.equal(self.iterations % self.steps_per_update, 0)
# Learning rate decay
lr = self.lr
if self.initial_decay > 0:
lr = lr * (1. / (1. + self.decay * K.cast(self.iterations,
K.dtype(self.decay))))
if not self.nesterov:
for p, g, m,sm in zip(params, grads,moments, sum_moments):
v = self.momentum*m - lr*g
# updates sum_moments, moments
self.updates.append(K.update(sm, sm+v))
self.updates.append(K.switch(cond1,K.update(m,sm/float(self.steps_per_update)),m))
new_p = p + m
# Apply constraint
if getattr(p, 'constraint', None) is not None:
new_p = p.constraint(new_p)
self.updates.append(K.switch(cond1,K.update(p,new_p),p))
# Clear up container
self.updates.append(K.switch(cond1,K.update(sm,K.zeros_like(sm)),sm))
return self.updates
Can anybody tell me why.
Thanks in advance.
tensorflow keras deep-learning
I'm using a tensorflow based keras to train a object detection network.
Due to small GPU memory size, have to update params once per some steps to "expand" batch size.
But my implementation can't successfully converge in Mask Rcnn training or perform badly comparing normal optimizers in simple cifar10 classification:
class custom_SGD(Optimizer):
def get_updates(self, loss, params):
"""Main params updates operation
"""
shapes = [K.int_shape(p) for p in params]
if self.nesterov:
sum_grads = [K.zeros(shape) for shape in shapes]
moments = [K.zeros(shape) for shape in shapes]
sum_moments = [K.zeros(shape) for shape in shapes]
# Current gradients
grads = self.get_gradients(loss, params)
self.updates = [K.update_add(self.iterations, 1)]
self.weights = [self.iterations] + moments
cond1 = K.equal(self.iterations % self.steps_per_update, 0)
# Learning rate decay
lr = self.lr
if self.initial_decay > 0:
lr = lr * (1. / (1. + self.decay * K.cast(self.iterations,
K.dtype(self.decay))))
if not self.nesterov:
for p, g, m,sm in zip(params, grads,moments, sum_moments):
v = self.momentum*m - lr*g
# updates sum_moments, moments
self.updates.append(K.update(sm, sm+v))
self.updates.append(K.switch(cond1,K.update(m,sm/float(self.steps_per_update)),m))
new_p = p + m
# Apply constraint
if getattr(p, 'constraint', None) is not None:
new_p = p.constraint(new_p)
self.updates.append(K.switch(cond1,K.update(p,new_p),p))
# Clear up container
self.updates.append(K.switch(cond1,K.update(sm,K.zeros_like(sm)),sm))
return self.updates
Can anybody tell me why.
Thanks in advance.
tensorflow keras deep-learning
tensorflow keras deep-learning
asked Nov 13 '18 at 3:58
Zhenshuo LiangZhenshuo Liang
64
64
add a comment |
add a comment |
0
active
oldest
votes
Your Answer
StackExchange.ifUsing("editor", function () {
StackExchange.using("externalEditor", function () {
StackExchange.using("snippets", function () {
StackExchange.snippets.init();
});
});
}, "code-snippets");
StackExchange.ready(function() {
var channelOptions = {
tags: "".split(" "),
id: "1"
};
initTagRenderer("".split(" "), "".split(" "), channelOptions);
StackExchange.using("externalEditor", function() {
// Have to fire editor after snippets, if snippets enabled
if (StackExchange.settings.snippets.snippetsEnabled) {
StackExchange.using("snippets", function() {
createEditor();
});
}
else {
createEditor();
}
});
function createEditor() {
StackExchange.prepareEditor({
heartbeatType: 'answer',
autoActivateHeartbeat: false,
convertImagesToLinks: true,
noModals: true,
showLowRepImageUploadWarning: true,
reputationToPostImages: 10,
bindNavPrevention: true,
postfix: "",
imageUploader: {
brandingHtml: "Powered by u003ca class="icon-imgur-white" href="https://imgur.com/"u003eu003c/au003e",
contentPolicyHtml: "User contributions licensed under u003ca href="https://creativecommons.org/licenses/by-sa/3.0/"u003ecc by-sa 3.0 with attribution requiredu003c/au003e u003ca href="https://stackoverflow.com/legal/content-policy"u003e(content policy)u003c/au003e",
allowUrls: true
},
onDemand: true,
discardSelector: ".discard-answer"
,immediatelyShowMarkdownHelp:true
});
}
});
Sign up or log in
StackExchange.ready(function () {
StackExchange.helpers.onClickDraftSave('#login-link');
});
Sign up using Google
Sign up using Facebook
Sign up using Email and Password
Post as a guest
Required, but never shown
StackExchange.ready(
function () {
StackExchange.openid.initPostLogin('.new-post-login', 'https%3a%2f%2fstackoverflow.com%2fquestions%2f53273568%2fhow-to-update-parameters-every-certain-steps%23new-answer', 'question_page');
}
);
Post as a guest
Required, but never shown
0
active
oldest
votes
0
active
oldest
votes
active
oldest
votes
active
oldest
votes
Thanks for contributing an answer to Stack Overflow!
- Please be sure to answer the question. Provide details and share your research!
But avoid …
- Asking for help, clarification, or responding to other answers.
- Making statements based on opinion; back them up with references or personal experience.
To learn more, see our tips on writing great answers.
Sign up or log in
StackExchange.ready(function () {
StackExchange.helpers.onClickDraftSave('#login-link');
});
Sign up using Google
Sign up using Facebook
Sign up using Email and Password
Post as a guest
Required, but never shown
StackExchange.ready(
function () {
StackExchange.openid.initPostLogin('.new-post-login', 'https%3a%2f%2fstackoverflow.com%2fquestions%2f53273568%2fhow-to-update-parameters-every-certain-steps%23new-answer', 'question_page');
}
);
Post as a guest
Required, but never shown
Sign up or log in
StackExchange.ready(function () {
StackExchange.helpers.onClickDraftSave('#login-link');
});
Sign up using Google
Sign up using Facebook
Sign up using Email and Password
Post as a guest
Required, but never shown
Sign up or log in
StackExchange.ready(function () {
StackExchange.helpers.onClickDraftSave('#login-link');
});
Sign up using Google
Sign up using Facebook
Sign up using Email and Password
Post as a guest
Required, but never shown
Sign up or log in
StackExchange.ready(function () {
StackExchange.helpers.onClickDraftSave('#login-link');
});
Sign up using Google
Sign up using Facebook
Sign up using Email and Password
Sign up using Google
Sign up using Facebook
Sign up using Email and Password
Post as a guest
Required, but never shown
Required, but never shown
Required, but never shown
Required, but never shown
Required, but never shown
Required, but never shown
Required, but never shown
Required, but never shown
Required, but never shown
BcPU 5Doz,Q VbweK54,HZeiyKX72LFBORqqBGn c,tzo,xtgsytUvBByo