implementation of optimistic greedy algorithm reinforcement learning
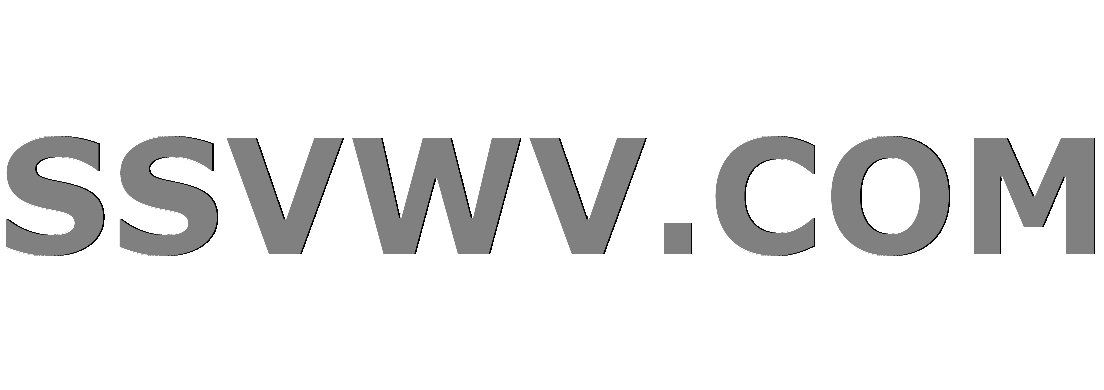
Multi tool use
up vote
-1
down vote
favorite
I been trying to implement optimistic greedy algorithm. My algorithm is able to find the optimal arm for the problem. but my results do not match with the quiz answers in the course. The R= 1 value should not find the optimal arm but my solution does.
I made a self.estimated_rewards array to store the initial reward value. (optimistic greedy algorithm needs a some initial rewards value setup). In the act function we just pull the arm which has max value. For first time it will pull the first arm as all arms have same value. but after each act() the feedback() function update the estimated_rewards array with new reward. I think the the problem might me in that last part or how i am updating the estimated_rewards array.
To see the full notebook I added the github link of this exercise also.
#Optimistic Greedy policy
class OptimisticGreedy(Greedy):
def __init__(self, num_actions, initial_value):
Greedy.__init__(self, num_actions)
self.name = "Optimistic Greedy"
# diy
"""Implement optimistic greedy here"""
self.total_rewards = np.zeros(num_actions, dtype = np.longdouble)
self.total_counts = np.zeros(num_actions, dtype = np.longdouble)
self.initial_value = initial_value
self.estimated_rewards = np.zeros(num_actions, dtype = np.longdouble)
self.estimated_rewards.fill(initial_value)
#diy
def act(self):
current_action = np.argmax(self.estimated_rewards)
#print(self.estimated_rewards)
#np.argmax(estimated_rewards)
return current_action
#diy
def feedback(self, action, reward):
self.total_rewards[action] += reward
self.total_counts[action] += 1
#self.estimated_rewards[action] = reward #updating the estimated rewards with actual reward recieved
self.estimated_rewards[action] = (reward+ self.estimated_rewards[action])/2 #updating the estimated rewards with actual reward received
course link:
https://courses.edx.org/courses/course-v1:Microsoft+DAT257x+2T2018
exercise link on github:
https://github.com/MicrosoftLearning/Reinforcement-Learning-Explained/blob/master/Module%202/Ex2.2B%20Optimistic%20Greedy.ipynb
(this course is not for credit)
python reinforcement-learning greedy openai-gym edx
add a comment |
up vote
-1
down vote
favorite
I been trying to implement optimistic greedy algorithm. My algorithm is able to find the optimal arm for the problem. but my results do not match with the quiz answers in the course. The R= 1 value should not find the optimal arm but my solution does.
I made a self.estimated_rewards array to store the initial reward value. (optimistic greedy algorithm needs a some initial rewards value setup). In the act function we just pull the arm which has max value. For first time it will pull the first arm as all arms have same value. but after each act() the feedback() function update the estimated_rewards array with new reward. I think the the problem might me in that last part or how i am updating the estimated_rewards array.
To see the full notebook I added the github link of this exercise also.
#Optimistic Greedy policy
class OptimisticGreedy(Greedy):
def __init__(self, num_actions, initial_value):
Greedy.__init__(self, num_actions)
self.name = "Optimistic Greedy"
# diy
"""Implement optimistic greedy here"""
self.total_rewards = np.zeros(num_actions, dtype = np.longdouble)
self.total_counts = np.zeros(num_actions, dtype = np.longdouble)
self.initial_value = initial_value
self.estimated_rewards = np.zeros(num_actions, dtype = np.longdouble)
self.estimated_rewards.fill(initial_value)
#diy
def act(self):
current_action = np.argmax(self.estimated_rewards)
#print(self.estimated_rewards)
#np.argmax(estimated_rewards)
return current_action
#diy
def feedback(self, action, reward):
self.total_rewards[action] += reward
self.total_counts[action] += 1
#self.estimated_rewards[action] = reward #updating the estimated rewards with actual reward recieved
self.estimated_rewards[action] = (reward+ self.estimated_rewards[action])/2 #updating the estimated rewards with actual reward received
course link:
https://courses.edx.org/courses/course-v1:Microsoft+DAT257x+2T2018
exercise link on github:
https://github.com/MicrosoftLearning/Reinforcement-Learning-Explained/blob/master/Module%202/Ex2.2B%20Optimistic%20Greedy.ipynb
(this course is not for credit)
python reinforcement-learning greedy openai-gym edx
add a comment |
up vote
-1
down vote
favorite
up vote
-1
down vote
favorite
I been trying to implement optimistic greedy algorithm. My algorithm is able to find the optimal arm for the problem. but my results do not match with the quiz answers in the course. The R= 1 value should not find the optimal arm but my solution does.
I made a self.estimated_rewards array to store the initial reward value. (optimistic greedy algorithm needs a some initial rewards value setup). In the act function we just pull the arm which has max value. For first time it will pull the first arm as all arms have same value. but after each act() the feedback() function update the estimated_rewards array with new reward. I think the the problem might me in that last part or how i am updating the estimated_rewards array.
To see the full notebook I added the github link of this exercise also.
#Optimistic Greedy policy
class OptimisticGreedy(Greedy):
def __init__(self, num_actions, initial_value):
Greedy.__init__(self, num_actions)
self.name = "Optimistic Greedy"
# diy
"""Implement optimistic greedy here"""
self.total_rewards = np.zeros(num_actions, dtype = np.longdouble)
self.total_counts = np.zeros(num_actions, dtype = np.longdouble)
self.initial_value = initial_value
self.estimated_rewards = np.zeros(num_actions, dtype = np.longdouble)
self.estimated_rewards.fill(initial_value)
#diy
def act(self):
current_action = np.argmax(self.estimated_rewards)
#print(self.estimated_rewards)
#np.argmax(estimated_rewards)
return current_action
#diy
def feedback(self, action, reward):
self.total_rewards[action] += reward
self.total_counts[action] += 1
#self.estimated_rewards[action] = reward #updating the estimated rewards with actual reward recieved
self.estimated_rewards[action] = (reward+ self.estimated_rewards[action])/2 #updating the estimated rewards with actual reward received
course link:
https://courses.edx.org/courses/course-v1:Microsoft+DAT257x+2T2018
exercise link on github:
https://github.com/MicrosoftLearning/Reinforcement-Learning-Explained/blob/master/Module%202/Ex2.2B%20Optimistic%20Greedy.ipynb
(this course is not for credit)
python reinforcement-learning greedy openai-gym edx
I been trying to implement optimistic greedy algorithm. My algorithm is able to find the optimal arm for the problem. but my results do not match with the quiz answers in the course. The R= 1 value should not find the optimal arm but my solution does.
I made a self.estimated_rewards array to store the initial reward value. (optimistic greedy algorithm needs a some initial rewards value setup). In the act function we just pull the arm which has max value. For first time it will pull the first arm as all arms have same value. but after each act() the feedback() function update the estimated_rewards array with new reward. I think the the problem might me in that last part or how i am updating the estimated_rewards array.
To see the full notebook I added the github link of this exercise also.
#Optimistic Greedy policy
class OptimisticGreedy(Greedy):
def __init__(self, num_actions, initial_value):
Greedy.__init__(self, num_actions)
self.name = "Optimistic Greedy"
# diy
"""Implement optimistic greedy here"""
self.total_rewards = np.zeros(num_actions, dtype = np.longdouble)
self.total_counts = np.zeros(num_actions, dtype = np.longdouble)
self.initial_value = initial_value
self.estimated_rewards = np.zeros(num_actions, dtype = np.longdouble)
self.estimated_rewards.fill(initial_value)
#diy
def act(self):
current_action = np.argmax(self.estimated_rewards)
#print(self.estimated_rewards)
#np.argmax(estimated_rewards)
return current_action
#diy
def feedback(self, action, reward):
self.total_rewards[action] += reward
self.total_counts[action] += 1
#self.estimated_rewards[action] = reward #updating the estimated rewards with actual reward recieved
self.estimated_rewards[action] = (reward+ self.estimated_rewards[action])/2 #updating the estimated rewards with actual reward received
course link:
https://courses.edx.org/courses/course-v1:Microsoft+DAT257x+2T2018
exercise link on github:
https://github.com/MicrosoftLearning/Reinforcement-Learning-Explained/blob/master/Module%202/Ex2.2B%20Optimistic%20Greedy.ipynb
(this course is not for credit)
python reinforcement-learning greedy openai-gym edx
python reinforcement-learning greedy openai-gym edx
asked Nov 10 at 18:23
shunya
79621330
79621330
add a comment |
add a comment |
active
oldest
votes
active
oldest
votes
active
oldest
votes
active
oldest
votes
active
oldest
votes
Sign up or log in
StackExchange.ready(function () {
StackExchange.helpers.onClickDraftSave('#login-link');
});
Sign up using Google
Sign up using Facebook
Sign up using Email and Password
Post as a guest
Required, but never shown
StackExchange.ready(
function () {
StackExchange.openid.initPostLogin('.new-post-login', 'https%3a%2f%2fstackoverflow.com%2fquestions%2f53242073%2fimplementation-of-optimistic-greedy-algorithm-reinforcement-learning%23new-answer', 'question_page');
}
);
Post as a guest
Required, but never shown
Sign up or log in
StackExchange.ready(function () {
StackExchange.helpers.onClickDraftSave('#login-link');
});
Sign up using Google
Sign up using Facebook
Sign up using Email and Password
Post as a guest
Required, but never shown
Sign up or log in
StackExchange.ready(function () {
StackExchange.helpers.onClickDraftSave('#login-link');
});
Sign up using Google
Sign up using Facebook
Sign up using Email and Password
Post as a guest
Required, but never shown
Sign up or log in
StackExchange.ready(function () {
StackExchange.helpers.onClickDraftSave('#login-link');
});
Sign up using Google
Sign up using Facebook
Sign up using Email and Password
Sign up using Google
Sign up using Facebook
Sign up using Email and Password
Post as a guest
Required, but never shown
Required, but never shown
Required, but never shown
Required, but never shown
Required, but never shown
Required, but never shown
Required, but never shown
Required, but never shown
Required, but never shown
ZmdMfl1rtbBI,JSf U,FVxt8 oQEhcR9fRc2iJzx dgfu16e8KI vwsOGR XwF H92ck swHQ3vQR7Eow5G,As3KsL,qOpko