Issue getting decision variable to optimize properly in Pyomo
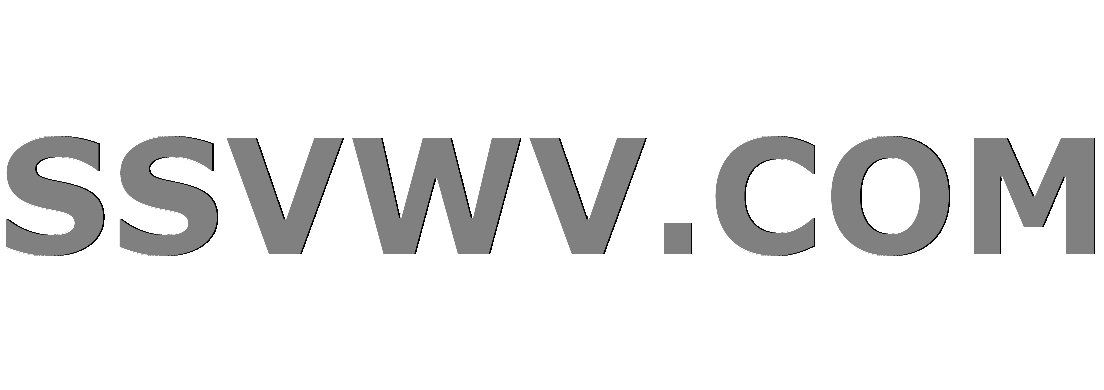
Multi tool use
I have a simple multi-period optimization problem I am working on using pyomo. The goal of the model is to determine which hours a power plant should be on or off based on the Spark Spread (Power Price - Gas Price * Heat Rate + Variable Costs) for that hour. The Spark spread can be negative, which would indicate that the plant should be off, or positive which means the plant should be running.
Currently the results are a showing that the plant just gets turned on and runs despite the spark spread being negative.
How can I get the plant to turn on and off at each time step, given the spark spread for that hour?
I am sure this is a fairly simple solution, but I am very new to pyomo and optimization problems, so any guidance and help would be much appreciated.
gas_price = [2.81,2.81,2.81,2.81,2.81,2.81,2.81,2.81,2.81,2.81,2.81,2.81,2.81,2.81,2.81,2.81,2.81]
power_price = [26.24,23.8,21.94,20.4,21.2,19.98,19.34,18.83,19.19,18.48,21,21.77,23.45,26.53,29.85,31.8,28.7]
priceDict = dict(enumerate(power_price))
gasDict = dict(enumerate(gas_price))
m = en.ConcreteModel()
m.Time = en.RangeSet(0, len(power_price)-1)
m.powerPrice = en.Param(m.Time, initialize=priceDict)
m.gasPrice = en.Param(m.Time, initialize=gasDict)
m.generation = en.Var(m.Time, bounds=(0,800),
initialize=0)
m.spark = en.Var(m.Time,initialize=0)
m.heatRate = en.Var(m.Time,initialize=7)
m.vom = en.Var(m.Time,initialize=2)
m.max_gen = en.Param(initialize=800)
def Obj_fn(m):
return sum((m.spark[i]*m.generation[i]) for i in m.Time)
m.total_cost = en.Objective(rule=Obj_fn,sense=en.maximize)
# 7 is the heat rate of the plant
def spark_rule(m,i):
return (m.spark[i] == m.powerPrice[i]-(m.gasPrice[i]*7+m.vom[i]))
m.hourly_spark = en.Constraint(m.Time,rule=spark_rule)
def generation_rule(m,i):
return (0<=m.generation[i]<=m.max_gen)
m.t_generation_rule = en.Constraint(m.Time, rule=generation_rule)
opt = SolverFactory("clp",executable='C:\clp.exe')
results = opt.solve(m)
The output of the model is currently:
Time Generation Spark Spread
1 0 6.57
2 800 4.13
3 800 2.27
4 800 0.73
5 800 1.53
6 800 0.31
7 800 -0.33
8 800 -0.84
9 800 -0.48
10 800 -1.19
11 800 1.33
12 800 2.1
13 800 3.78
14 800 6.86
15 800 10.18
16 800 12.13
17 800 9.03
python optimization pyomo glpk clp
|
show 1 more comment
I have a simple multi-period optimization problem I am working on using pyomo. The goal of the model is to determine which hours a power plant should be on or off based on the Spark Spread (Power Price - Gas Price * Heat Rate + Variable Costs) for that hour. The Spark spread can be negative, which would indicate that the plant should be off, or positive which means the plant should be running.
Currently the results are a showing that the plant just gets turned on and runs despite the spark spread being negative.
How can I get the plant to turn on and off at each time step, given the spark spread for that hour?
I am sure this is a fairly simple solution, but I am very new to pyomo and optimization problems, so any guidance and help would be much appreciated.
gas_price = [2.81,2.81,2.81,2.81,2.81,2.81,2.81,2.81,2.81,2.81,2.81,2.81,2.81,2.81,2.81,2.81,2.81]
power_price = [26.24,23.8,21.94,20.4,21.2,19.98,19.34,18.83,19.19,18.48,21,21.77,23.45,26.53,29.85,31.8,28.7]
priceDict = dict(enumerate(power_price))
gasDict = dict(enumerate(gas_price))
m = en.ConcreteModel()
m.Time = en.RangeSet(0, len(power_price)-1)
m.powerPrice = en.Param(m.Time, initialize=priceDict)
m.gasPrice = en.Param(m.Time, initialize=gasDict)
m.generation = en.Var(m.Time, bounds=(0,800),
initialize=0)
m.spark = en.Var(m.Time,initialize=0)
m.heatRate = en.Var(m.Time,initialize=7)
m.vom = en.Var(m.Time,initialize=2)
m.max_gen = en.Param(initialize=800)
def Obj_fn(m):
return sum((m.spark[i]*m.generation[i]) for i in m.Time)
m.total_cost = en.Objective(rule=Obj_fn,sense=en.maximize)
# 7 is the heat rate of the plant
def spark_rule(m,i):
return (m.spark[i] == m.powerPrice[i]-(m.gasPrice[i]*7+m.vom[i]))
m.hourly_spark = en.Constraint(m.Time,rule=spark_rule)
def generation_rule(m,i):
return (0<=m.generation[i]<=m.max_gen)
m.t_generation_rule = en.Constraint(m.Time, rule=generation_rule)
opt = SolverFactory("clp",executable='C:\clp.exe')
results = opt.solve(m)
The output of the model is currently:
Time Generation Spark Spread
1 0 6.57
2 800 4.13
3 800 2.27
4 800 0.73
5 800 1.53
6 800 0.31
7 800 -0.33
8 800 -0.84
9 800 -0.48
10 800 -1.19
11 800 1.33
12 800 2.1
13 800 3.78
14 800 6.86
15 800 10.18
16 800 12.13
17 800 9.03
python optimization pyomo glpk clp
Not sure I follow, but don´t you want to optimize sum((max(m.spark[i, 0)]*m.generation[i]) for i in m.Time) so that when spark is negative the plant should not run -> not add generation?
– juvian
Nov 12 '18 at 18:47
Well the model should evaluate the spark spread at each time step and decide if the 'plant' should be generating in that hour or off in that hour. My thinking was that by maximizing the sum of generation * spark spread, the optimization would decide when generation should be 0 or 800
– mgeoffb
Nov 12 '18 at 19:30
Could you add the values of the current solution it is showing?
– juvian
Nov 12 '18 at 21:28
This looks like a nonconvex QP. They are not so easy to solve. You need a global solver for that. I believe CLP only supports convex QPs.
– Erwin Kalvelagen
Nov 13 '18 at 6:00
Thanks for the thoughts @ErwinKalvelagen, I may try and reformulate the problem so I can use a solver such as GLPK/Clp.
– mgeoffb
Nov 13 '18 at 22:06
|
show 1 more comment
I have a simple multi-period optimization problem I am working on using pyomo. The goal of the model is to determine which hours a power plant should be on or off based on the Spark Spread (Power Price - Gas Price * Heat Rate + Variable Costs) for that hour. The Spark spread can be negative, which would indicate that the plant should be off, or positive which means the plant should be running.
Currently the results are a showing that the plant just gets turned on and runs despite the spark spread being negative.
How can I get the plant to turn on and off at each time step, given the spark spread for that hour?
I am sure this is a fairly simple solution, but I am very new to pyomo and optimization problems, so any guidance and help would be much appreciated.
gas_price = [2.81,2.81,2.81,2.81,2.81,2.81,2.81,2.81,2.81,2.81,2.81,2.81,2.81,2.81,2.81,2.81,2.81]
power_price = [26.24,23.8,21.94,20.4,21.2,19.98,19.34,18.83,19.19,18.48,21,21.77,23.45,26.53,29.85,31.8,28.7]
priceDict = dict(enumerate(power_price))
gasDict = dict(enumerate(gas_price))
m = en.ConcreteModel()
m.Time = en.RangeSet(0, len(power_price)-1)
m.powerPrice = en.Param(m.Time, initialize=priceDict)
m.gasPrice = en.Param(m.Time, initialize=gasDict)
m.generation = en.Var(m.Time, bounds=(0,800),
initialize=0)
m.spark = en.Var(m.Time,initialize=0)
m.heatRate = en.Var(m.Time,initialize=7)
m.vom = en.Var(m.Time,initialize=2)
m.max_gen = en.Param(initialize=800)
def Obj_fn(m):
return sum((m.spark[i]*m.generation[i]) for i in m.Time)
m.total_cost = en.Objective(rule=Obj_fn,sense=en.maximize)
# 7 is the heat rate of the plant
def spark_rule(m,i):
return (m.spark[i] == m.powerPrice[i]-(m.gasPrice[i]*7+m.vom[i]))
m.hourly_spark = en.Constraint(m.Time,rule=spark_rule)
def generation_rule(m,i):
return (0<=m.generation[i]<=m.max_gen)
m.t_generation_rule = en.Constraint(m.Time, rule=generation_rule)
opt = SolverFactory("clp",executable='C:\clp.exe')
results = opt.solve(m)
The output of the model is currently:
Time Generation Spark Spread
1 0 6.57
2 800 4.13
3 800 2.27
4 800 0.73
5 800 1.53
6 800 0.31
7 800 -0.33
8 800 -0.84
9 800 -0.48
10 800 -1.19
11 800 1.33
12 800 2.1
13 800 3.78
14 800 6.86
15 800 10.18
16 800 12.13
17 800 9.03
python optimization pyomo glpk clp
I have a simple multi-period optimization problem I am working on using pyomo. The goal of the model is to determine which hours a power plant should be on or off based on the Spark Spread (Power Price - Gas Price * Heat Rate + Variable Costs) for that hour. The Spark spread can be negative, which would indicate that the plant should be off, or positive which means the plant should be running.
Currently the results are a showing that the plant just gets turned on and runs despite the spark spread being negative.
How can I get the plant to turn on and off at each time step, given the spark spread for that hour?
I am sure this is a fairly simple solution, but I am very new to pyomo and optimization problems, so any guidance and help would be much appreciated.
gas_price = [2.81,2.81,2.81,2.81,2.81,2.81,2.81,2.81,2.81,2.81,2.81,2.81,2.81,2.81,2.81,2.81,2.81]
power_price = [26.24,23.8,21.94,20.4,21.2,19.98,19.34,18.83,19.19,18.48,21,21.77,23.45,26.53,29.85,31.8,28.7]
priceDict = dict(enumerate(power_price))
gasDict = dict(enumerate(gas_price))
m = en.ConcreteModel()
m.Time = en.RangeSet(0, len(power_price)-1)
m.powerPrice = en.Param(m.Time, initialize=priceDict)
m.gasPrice = en.Param(m.Time, initialize=gasDict)
m.generation = en.Var(m.Time, bounds=(0,800),
initialize=0)
m.spark = en.Var(m.Time,initialize=0)
m.heatRate = en.Var(m.Time,initialize=7)
m.vom = en.Var(m.Time,initialize=2)
m.max_gen = en.Param(initialize=800)
def Obj_fn(m):
return sum((m.spark[i]*m.generation[i]) for i in m.Time)
m.total_cost = en.Objective(rule=Obj_fn,sense=en.maximize)
# 7 is the heat rate of the plant
def spark_rule(m,i):
return (m.spark[i] == m.powerPrice[i]-(m.gasPrice[i]*7+m.vom[i]))
m.hourly_spark = en.Constraint(m.Time,rule=spark_rule)
def generation_rule(m,i):
return (0<=m.generation[i]<=m.max_gen)
m.t_generation_rule = en.Constraint(m.Time, rule=generation_rule)
opt = SolverFactory("clp",executable='C:\clp.exe')
results = opt.solve(m)
The output of the model is currently:
Time Generation Spark Spread
1 0 6.57
2 800 4.13
3 800 2.27
4 800 0.73
5 800 1.53
6 800 0.31
7 800 -0.33
8 800 -0.84
9 800 -0.48
10 800 -1.19
11 800 1.33
12 800 2.1
13 800 3.78
14 800 6.86
15 800 10.18
16 800 12.13
17 800 9.03
python optimization pyomo glpk clp
python optimization pyomo glpk clp
edited Nov 12 '18 at 22:19
asked Nov 12 '18 at 18:21
mgeoffb
11
11
Not sure I follow, but don´t you want to optimize sum((max(m.spark[i, 0)]*m.generation[i]) for i in m.Time) so that when spark is negative the plant should not run -> not add generation?
– juvian
Nov 12 '18 at 18:47
Well the model should evaluate the spark spread at each time step and decide if the 'plant' should be generating in that hour or off in that hour. My thinking was that by maximizing the sum of generation * spark spread, the optimization would decide when generation should be 0 or 800
– mgeoffb
Nov 12 '18 at 19:30
Could you add the values of the current solution it is showing?
– juvian
Nov 12 '18 at 21:28
This looks like a nonconvex QP. They are not so easy to solve. You need a global solver for that. I believe CLP only supports convex QPs.
– Erwin Kalvelagen
Nov 13 '18 at 6:00
Thanks for the thoughts @ErwinKalvelagen, I may try and reformulate the problem so I can use a solver such as GLPK/Clp.
– mgeoffb
Nov 13 '18 at 22:06
|
show 1 more comment
Not sure I follow, but don´t you want to optimize sum((max(m.spark[i, 0)]*m.generation[i]) for i in m.Time) so that when spark is negative the plant should not run -> not add generation?
– juvian
Nov 12 '18 at 18:47
Well the model should evaluate the spark spread at each time step and decide if the 'plant' should be generating in that hour or off in that hour. My thinking was that by maximizing the sum of generation * spark spread, the optimization would decide when generation should be 0 or 800
– mgeoffb
Nov 12 '18 at 19:30
Could you add the values of the current solution it is showing?
– juvian
Nov 12 '18 at 21:28
This looks like a nonconvex QP. They are not so easy to solve. You need a global solver for that. I believe CLP only supports convex QPs.
– Erwin Kalvelagen
Nov 13 '18 at 6:00
Thanks for the thoughts @ErwinKalvelagen, I may try and reformulate the problem so I can use a solver such as GLPK/Clp.
– mgeoffb
Nov 13 '18 at 22:06
Not sure I follow, but don´t you want to optimize sum((max(m.spark[i, 0)]*m.generation[i]) for i in m.Time) so that when spark is negative the plant should not run -> not add generation?
– juvian
Nov 12 '18 at 18:47
Not sure I follow, but don´t you want to optimize sum((max(m.spark[i, 0)]*m.generation[i]) for i in m.Time) so that when spark is negative the plant should not run -> not add generation?
– juvian
Nov 12 '18 at 18:47
Well the model should evaluate the spark spread at each time step and decide if the 'plant' should be generating in that hour or off in that hour. My thinking was that by maximizing the sum of generation * spark spread, the optimization would decide when generation should be 0 or 800
– mgeoffb
Nov 12 '18 at 19:30
Well the model should evaluate the spark spread at each time step and decide if the 'plant' should be generating in that hour or off in that hour. My thinking was that by maximizing the sum of generation * spark spread, the optimization would decide when generation should be 0 or 800
– mgeoffb
Nov 12 '18 at 19:30
Could you add the values of the current solution it is showing?
– juvian
Nov 12 '18 at 21:28
Could you add the values of the current solution it is showing?
– juvian
Nov 12 '18 at 21:28
This looks like a nonconvex QP. They are not so easy to solve. You need a global solver for that. I believe CLP only supports convex QPs.
– Erwin Kalvelagen
Nov 13 '18 at 6:00
This looks like a nonconvex QP. They are not so easy to solve. You need a global solver for that. I believe CLP only supports convex QPs.
– Erwin Kalvelagen
Nov 13 '18 at 6:00
Thanks for the thoughts @ErwinKalvelagen, I may try and reformulate the problem so I can use a solver such as GLPK/Clp.
– mgeoffb
Nov 13 '18 at 22:06
Thanks for the thoughts @ErwinKalvelagen, I may try and reformulate the problem so I can use a solver such as GLPK/Clp.
– mgeoffb
Nov 13 '18 at 22:06
|
show 1 more comment
1 Answer
1
active
oldest
votes
I might be wrong here, but I think you actually meant to define heatRate
and vom
as parameters, rather than variables.
This leads to a weird problem because the plant will naturally use its max power whenever the "spark" price is positive, and go to 0 whenever the spark price is negative. I guess you'll add more constraints later on.
If heatRate
and vom
are fixed, then you can redefine the problem in the following way:
from pyomo import environ as pe
gas_price = [2.81,2.81,2.81,2.81,2.81,2.81,2.81,2.81,2.81,2.81,2.81,2.81,2.81,2.81,2.81,2.81,2.81]
power_price = [26.24,23.8,21.94,20.4,21.2,19.98,19.34,18.83,19.19,18.48,21,21.77,23.45,26.53,29.85,31.8,28.7]
priceDict = dict(enumerate(power_price))
gasDict = dict(enumerate(gas_price))
m = pe.ConcreteModel()
m.Time = pe.RangeSet(0, len(power_price)-1)
# this are all input parameters
m.powerPrice = pe.Param(m.Time, initialize=priceDict)
m.gasPrice = pe.Param(m.Time, initialize=gasDict)
m.vom = pe.Param(default=7)
m.heatRate = pe.Param(default=2)
m.maxGen = pe.Param(default=800)
# this is a "dependent" parameter
m.spark = pe.Param(m.Time,
initialize = lambda m,t: m.powerPrice[t]-(m.gasPrice[t]*7+m.vom)
)
# this is the only variable
m.generation = pe.Var(m.Time,
initialize=0,
bounds = (0, m.maxGen)
)
def Obj_fn(m):
return sum((m.spark[t]*m.generation[t]) for t in m.Time)
m.total_cost = pe.Objective(rule=Obj_fn,sense=pe.maximize)
add a comment |
Your Answer
StackExchange.ifUsing("editor", function () {
StackExchange.using("externalEditor", function () {
StackExchange.using("snippets", function () {
StackExchange.snippets.init();
});
});
}, "code-snippets");
StackExchange.ready(function() {
var channelOptions = {
tags: "".split(" "),
id: "1"
};
initTagRenderer("".split(" "), "".split(" "), channelOptions);
StackExchange.using("externalEditor", function() {
// Have to fire editor after snippets, if snippets enabled
if (StackExchange.settings.snippets.snippetsEnabled) {
StackExchange.using("snippets", function() {
createEditor();
});
}
else {
createEditor();
}
});
function createEditor() {
StackExchange.prepareEditor({
heartbeatType: 'answer',
autoActivateHeartbeat: false,
convertImagesToLinks: true,
noModals: true,
showLowRepImageUploadWarning: true,
reputationToPostImages: 10,
bindNavPrevention: true,
postfix: "",
imageUploader: {
brandingHtml: "Powered by u003ca class="icon-imgur-white" href="https://imgur.com/"u003eu003c/au003e",
contentPolicyHtml: "User contributions licensed under u003ca href="https://creativecommons.org/licenses/by-sa/3.0/"u003ecc by-sa 3.0 with attribution requiredu003c/au003e u003ca href="https://stackoverflow.com/legal/content-policy"u003e(content policy)u003c/au003e",
allowUrls: true
},
onDemand: true,
discardSelector: ".discard-answer"
,immediatelyShowMarkdownHelp:true
});
}
});
Sign up or log in
StackExchange.ready(function () {
StackExchange.helpers.onClickDraftSave('#login-link');
});
Sign up using Google
Sign up using Facebook
Sign up using Email and Password
Post as a guest
Required, but never shown
StackExchange.ready(
function () {
StackExchange.openid.initPostLogin('.new-post-login', 'https%3a%2f%2fstackoverflow.com%2fquestions%2f53267963%2fissue-getting-decision-variable-to-optimize-properly-in-pyomo%23new-answer', 'question_page');
}
);
Post as a guest
Required, but never shown
1 Answer
1
active
oldest
votes
1 Answer
1
active
oldest
votes
active
oldest
votes
active
oldest
votes
I might be wrong here, but I think you actually meant to define heatRate
and vom
as parameters, rather than variables.
This leads to a weird problem because the plant will naturally use its max power whenever the "spark" price is positive, and go to 0 whenever the spark price is negative. I guess you'll add more constraints later on.
If heatRate
and vom
are fixed, then you can redefine the problem in the following way:
from pyomo import environ as pe
gas_price = [2.81,2.81,2.81,2.81,2.81,2.81,2.81,2.81,2.81,2.81,2.81,2.81,2.81,2.81,2.81,2.81,2.81]
power_price = [26.24,23.8,21.94,20.4,21.2,19.98,19.34,18.83,19.19,18.48,21,21.77,23.45,26.53,29.85,31.8,28.7]
priceDict = dict(enumerate(power_price))
gasDict = dict(enumerate(gas_price))
m = pe.ConcreteModel()
m.Time = pe.RangeSet(0, len(power_price)-1)
# this are all input parameters
m.powerPrice = pe.Param(m.Time, initialize=priceDict)
m.gasPrice = pe.Param(m.Time, initialize=gasDict)
m.vom = pe.Param(default=7)
m.heatRate = pe.Param(default=2)
m.maxGen = pe.Param(default=800)
# this is a "dependent" parameter
m.spark = pe.Param(m.Time,
initialize = lambda m,t: m.powerPrice[t]-(m.gasPrice[t]*7+m.vom)
)
# this is the only variable
m.generation = pe.Var(m.Time,
initialize=0,
bounds = (0, m.maxGen)
)
def Obj_fn(m):
return sum((m.spark[t]*m.generation[t]) for t in m.Time)
m.total_cost = pe.Objective(rule=Obj_fn,sense=pe.maximize)
add a comment |
I might be wrong here, but I think you actually meant to define heatRate
and vom
as parameters, rather than variables.
This leads to a weird problem because the plant will naturally use its max power whenever the "spark" price is positive, and go to 0 whenever the spark price is negative. I guess you'll add more constraints later on.
If heatRate
and vom
are fixed, then you can redefine the problem in the following way:
from pyomo import environ as pe
gas_price = [2.81,2.81,2.81,2.81,2.81,2.81,2.81,2.81,2.81,2.81,2.81,2.81,2.81,2.81,2.81,2.81,2.81]
power_price = [26.24,23.8,21.94,20.4,21.2,19.98,19.34,18.83,19.19,18.48,21,21.77,23.45,26.53,29.85,31.8,28.7]
priceDict = dict(enumerate(power_price))
gasDict = dict(enumerate(gas_price))
m = pe.ConcreteModel()
m.Time = pe.RangeSet(0, len(power_price)-1)
# this are all input parameters
m.powerPrice = pe.Param(m.Time, initialize=priceDict)
m.gasPrice = pe.Param(m.Time, initialize=gasDict)
m.vom = pe.Param(default=7)
m.heatRate = pe.Param(default=2)
m.maxGen = pe.Param(default=800)
# this is a "dependent" parameter
m.spark = pe.Param(m.Time,
initialize = lambda m,t: m.powerPrice[t]-(m.gasPrice[t]*7+m.vom)
)
# this is the only variable
m.generation = pe.Var(m.Time,
initialize=0,
bounds = (0, m.maxGen)
)
def Obj_fn(m):
return sum((m.spark[t]*m.generation[t]) for t in m.Time)
m.total_cost = pe.Objective(rule=Obj_fn,sense=pe.maximize)
add a comment |
I might be wrong here, but I think you actually meant to define heatRate
and vom
as parameters, rather than variables.
This leads to a weird problem because the plant will naturally use its max power whenever the "spark" price is positive, and go to 0 whenever the spark price is negative. I guess you'll add more constraints later on.
If heatRate
and vom
are fixed, then you can redefine the problem in the following way:
from pyomo import environ as pe
gas_price = [2.81,2.81,2.81,2.81,2.81,2.81,2.81,2.81,2.81,2.81,2.81,2.81,2.81,2.81,2.81,2.81,2.81]
power_price = [26.24,23.8,21.94,20.4,21.2,19.98,19.34,18.83,19.19,18.48,21,21.77,23.45,26.53,29.85,31.8,28.7]
priceDict = dict(enumerate(power_price))
gasDict = dict(enumerate(gas_price))
m = pe.ConcreteModel()
m.Time = pe.RangeSet(0, len(power_price)-1)
# this are all input parameters
m.powerPrice = pe.Param(m.Time, initialize=priceDict)
m.gasPrice = pe.Param(m.Time, initialize=gasDict)
m.vom = pe.Param(default=7)
m.heatRate = pe.Param(default=2)
m.maxGen = pe.Param(default=800)
# this is a "dependent" parameter
m.spark = pe.Param(m.Time,
initialize = lambda m,t: m.powerPrice[t]-(m.gasPrice[t]*7+m.vom)
)
# this is the only variable
m.generation = pe.Var(m.Time,
initialize=0,
bounds = (0, m.maxGen)
)
def Obj_fn(m):
return sum((m.spark[t]*m.generation[t]) for t in m.Time)
m.total_cost = pe.Objective(rule=Obj_fn,sense=pe.maximize)
I might be wrong here, but I think you actually meant to define heatRate
and vom
as parameters, rather than variables.
This leads to a weird problem because the plant will naturally use its max power whenever the "spark" price is positive, and go to 0 whenever the spark price is negative. I guess you'll add more constraints later on.
If heatRate
and vom
are fixed, then you can redefine the problem in the following way:
from pyomo import environ as pe
gas_price = [2.81,2.81,2.81,2.81,2.81,2.81,2.81,2.81,2.81,2.81,2.81,2.81,2.81,2.81,2.81,2.81,2.81]
power_price = [26.24,23.8,21.94,20.4,21.2,19.98,19.34,18.83,19.19,18.48,21,21.77,23.45,26.53,29.85,31.8,28.7]
priceDict = dict(enumerate(power_price))
gasDict = dict(enumerate(gas_price))
m = pe.ConcreteModel()
m.Time = pe.RangeSet(0, len(power_price)-1)
# this are all input parameters
m.powerPrice = pe.Param(m.Time, initialize=priceDict)
m.gasPrice = pe.Param(m.Time, initialize=gasDict)
m.vom = pe.Param(default=7)
m.heatRate = pe.Param(default=2)
m.maxGen = pe.Param(default=800)
# this is a "dependent" parameter
m.spark = pe.Param(m.Time,
initialize = lambda m,t: m.powerPrice[t]-(m.gasPrice[t]*7+m.vom)
)
# this is the only variable
m.generation = pe.Var(m.Time,
initialize=0,
bounds = (0, m.maxGen)
)
def Obj_fn(m):
return sum((m.spark[t]*m.generation[t]) for t in m.Time)
m.total_cost = pe.Objective(rule=Obj_fn,sense=pe.maximize)
answered Nov 21 '18 at 1:26


Giorgio Balestrieri
645
645
add a comment |
add a comment |
Thanks for contributing an answer to Stack Overflow!
- Please be sure to answer the question. Provide details and share your research!
But avoid …
- Asking for help, clarification, or responding to other answers.
- Making statements based on opinion; back them up with references or personal experience.
To learn more, see our tips on writing great answers.
Some of your past answers have not been well-received, and you're in danger of being blocked from answering.
Please pay close attention to the following guidance:
- Please be sure to answer the question. Provide details and share your research!
But avoid …
- Asking for help, clarification, or responding to other answers.
- Making statements based on opinion; back them up with references or personal experience.
To learn more, see our tips on writing great answers.
Sign up or log in
StackExchange.ready(function () {
StackExchange.helpers.onClickDraftSave('#login-link');
});
Sign up using Google
Sign up using Facebook
Sign up using Email and Password
Post as a guest
Required, but never shown
StackExchange.ready(
function () {
StackExchange.openid.initPostLogin('.new-post-login', 'https%3a%2f%2fstackoverflow.com%2fquestions%2f53267963%2fissue-getting-decision-variable-to-optimize-properly-in-pyomo%23new-answer', 'question_page');
}
);
Post as a guest
Required, but never shown
Sign up or log in
StackExchange.ready(function () {
StackExchange.helpers.onClickDraftSave('#login-link');
});
Sign up using Google
Sign up using Facebook
Sign up using Email and Password
Post as a guest
Required, but never shown
Sign up or log in
StackExchange.ready(function () {
StackExchange.helpers.onClickDraftSave('#login-link');
});
Sign up using Google
Sign up using Facebook
Sign up using Email and Password
Post as a guest
Required, but never shown
Sign up or log in
StackExchange.ready(function () {
StackExchange.helpers.onClickDraftSave('#login-link');
});
Sign up using Google
Sign up using Facebook
Sign up using Email and Password
Sign up using Google
Sign up using Facebook
Sign up using Email and Password
Post as a guest
Required, but never shown
Required, but never shown
Required, but never shown
Required, but never shown
Required, but never shown
Required, but never shown
Required, but never shown
Required, but never shown
Required, but never shown
jHtiBYa E51Pe9 oDIu jo bCG4K,8MxCBnNgfTo2,0
Not sure I follow, but don´t you want to optimize sum((max(m.spark[i, 0)]*m.generation[i]) for i in m.Time) so that when spark is negative the plant should not run -> not add generation?
– juvian
Nov 12 '18 at 18:47
Well the model should evaluate the spark spread at each time step and decide if the 'plant' should be generating in that hour or off in that hour. My thinking was that by maximizing the sum of generation * spark spread, the optimization would decide when generation should be 0 or 800
– mgeoffb
Nov 12 '18 at 19:30
Could you add the values of the current solution it is showing?
– juvian
Nov 12 '18 at 21:28
This looks like a nonconvex QP. They are not so easy to solve. You need a global solver for that. I believe CLP only supports convex QPs.
– Erwin Kalvelagen
Nov 13 '18 at 6:00
Thanks for the thoughts @ErwinKalvelagen, I may try and reformulate the problem so I can use a solver such as GLPK/Clp.
– mgeoffb
Nov 13 '18 at 22:06