TSQL: calculate linear weighted (moving) average on a discrete time series
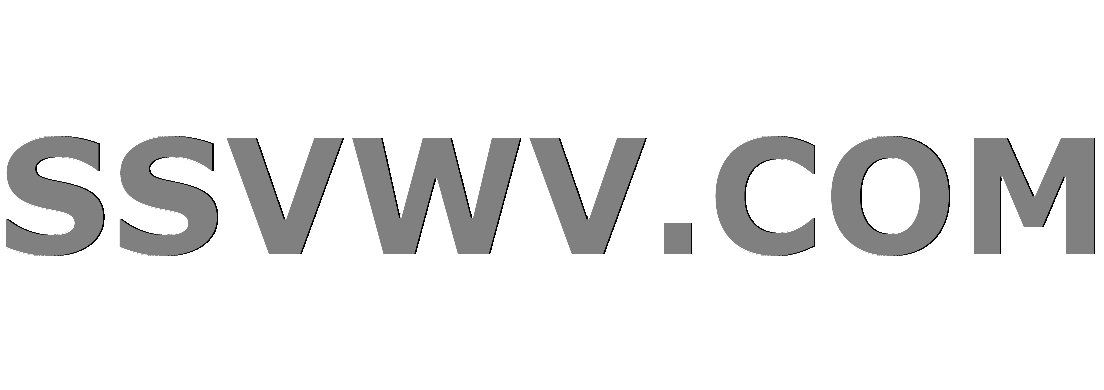
Multi tool use
up vote
0
down vote
favorite
I have a discrete time series which looks as follows:
product_id date sales_per_day
VSG19 2018-05-19 1.00000000000000
VSG19 2018-05-23 1.00000000000000
VSG19 2018-05-24 2.00000000000000
VSG19 2018-06-25 1.00000000000000
VSG19 2018-07-26 1.00000000000000
VSG19 2018-07-28 1.00000000000000
VSG19 2018-08-01 1.00000000000000
VSG19 2018-08-11 1.00000000000000
VSG19 2018-08-29 1.00000000000000
VSG19 2018-09-11 1.00000000000000
VSG19 2018-09-29 1.00000000000000
VSG19 2018-10-16 1.00000000000000
VSG19 2018-10-25 1.00000000000000
VSG19 2018-11-02 1.00000000000000
I'd like to calculate the linear weighted average for this, but my data does not contain days where no sale occured.
I have solved it by joining a calendar table, but I don't like this solution.
Do you know an elegant way to solve this?
Thanks in advance!
PS - Here's the formula for the LWMA: https://en.wikipedia.org/wiki/Moving_average#Weighted_moving_average


add a comment |
up vote
0
down vote
favorite
I have a discrete time series which looks as follows:
product_id date sales_per_day
VSG19 2018-05-19 1.00000000000000
VSG19 2018-05-23 1.00000000000000
VSG19 2018-05-24 2.00000000000000
VSG19 2018-06-25 1.00000000000000
VSG19 2018-07-26 1.00000000000000
VSG19 2018-07-28 1.00000000000000
VSG19 2018-08-01 1.00000000000000
VSG19 2018-08-11 1.00000000000000
VSG19 2018-08-29 1.00000000000000
VSG19 2018-09-11 1.00000000000000
VSG19 2018-09-29 1.00000000000000
VSG19 2018-10-16 1.00000000000000
VSG19 2018-10-25 1.00000000000000
VSG19 2018-11-02 1.00000000000000
I'd like to calculate the linear weighted average for this, but my data does not contain days where no sale occured.
I have solved it by joining a calendar table, but I don't like this solution.
Do you know an elegant way to solve this?
Thanks in advance!
PS - Here's the formula for the LWMA: https://en.wikipedia.org/wiki/Moving_average#Weighted_moving_average


3
What's wrong with a calendar table? I would suggest that that is the normal (and elegant?) way to do so.
– Larnu
Nov 11 at 19:55
@Larnu is correct - that is the correct and elegant solution.
– Dale Burrell
Nov 11 at 20:18
add a comment |
up vote
0
down vote
favorite
up vote
0
down vote
favorite
I have a discrete time series which looks as follows:
product_id date sales_per_day
VSG19 2018-05-19 1.00000000000000
VSG19 2018-05-23 1.00000000000000
VSG19 2018-05-24 2.00000000000000
VSG19 2018-06-25 1.00000000000000
VSG19 2018-07-26 1.00000000000000
VSG19 2018-07-28 1.00000000000000
VSG19 2018-08-01 1.00000000000000
VSG19 2018-08-11 1.00000000000000
VSG19 2018-08-29 1.00000000000000
VSG19 2018-09-11 1.00000000000000
VSG19 2018-09-29 1.00000000000000
VSG19 2018-10-16 1.00000000000000
VSG19 2018-10-25 1.00000000000000
VSG19 2018-11-02 1.00000000000000
I'd like to calculate the linear weighted average for this, but my data does not contain days where no sale occured.
I have solved it by joining a calendar table, but I don't like this solution.
Do you know an elegant way to solve this?
Thanks in advance!
PS - Here's the formula for the LWMA: https://en.wikipedia.org/wiki/Moving_average#Weighted_moving_average


I have a discrete time series which looks as follows:
product_id date sales_per_day
VSG19 2018-05-19 1.00000000000000
VSG19 2018-05-23 1.00000000000000
VSG19 2018-05-24 2.00000000000000
VSG19 2018-06-25 1.00000000000000
VSG19 2018-07-26 1.00000000000000
VSG19 2018-07-28 1.00000000000000
VSG19 2018-08-01 1.00000000000000
VSG19 2018-08-11 1.00000000000000
VSG19 2018-08-29 1.00000000000000
VSG19 2018-09-11 1.00000000000000
VSG19 2018-09-29 1.00000000000000
VSG19 2018-10-16 1.00000000000000
VSG19 2018-10-25 1.00000000000000
VSG19 2018-11-02 1.00000000000000
I'd like to calculate the linear weighted average for this, but my data does not contain days where no sale occured.
I have solved it by joining a calendar table, but I don't like this solution.
Do you know an elegant way to solve this?
Thanks in advance!
PS - Here's the formula for the LWMA: https://en.wikipedia.org/wiki/Moving_average#Weighted_moving_average




asked Nov 11 at 19:49
youvebeennarddogged
82
82
3
What's wrong with a calendar table? I would suggest that that is the normal (and elegant?) way to do so.
– Larnu
Nov 11 at 19:55
@Larnu is correct - that is the correct and elegant solution.
– Dale Burrell
Nov 11 at 20:18
add a comment |
3
What's wrong with a calendar table? I would suggest that that is the normal (and elegant?) way to do so.
– Larnu
Nov 11 at 19:55
@Larnu is correct - that is the correct and elegant solution.
– Dale Burrell
Nov 11 at 20:18
3
3
What's wrong with a calendar table? I would suggest that that is the normal (and elegant?) way to do so.
– Larnu
Nov 11 at 19:55
What's wrong with a calendar table? I would suggest that that is the normal (and elegant?) way to do so.
– Larnu
Nov 11 at 19:55
@Larnu is correct - that is the correct and elegant solution.
– Dale Burrell
Nov 11 at 20:18
@Larnu is correct - that is the correct and elegant solution.
– Dale Burrell
Nov 11 at 20:18
add a comment |
1 Answer
1
active
oldest
votes
up vote
0
down vote
I'm not overly familiar with that particular calculation but from what I just read, you should be able to use "window frames" to calculate the "rolling information need to assign the weights to past sales.
Without seeing the actual formula you're applying, I can't say for sure weather or not it will work or not.
The following is just an example off the top of my head...
IF OBJECT_ID('tempdb..#TestData', 'U') IS NOT NULL
DROP TABLE #TestData;
CREATE TABLE #TestData (
product_id CHAR(5) NOT NULL,
[date] DATE NOT NULL,
sales_per_day DECIMAL(19,14) NOT NULL
);
INSERT #TestData (product_id, date, sales_per_day) VALUES
('VSG19', '2018-05-19', 1.00000000000000),
('VSG19', '2018-05-23', 1.00000000000000),
('VSG19', '2018-05-24', 2.00000000000000),
('VSG19', '2018-06-25', 1.00000000000000),
('VSG19', '2018-07-26', 1.00000000000000),
('VSG19', '2018-07-28', 1.00000000000000),
('VSG19', '2018-08-01', 1.00000000000000),
('VSG19', '2018-08-11', 1.00000000000000),
('VSG19', '2018-08-29', 1.00000000000000),
('VSG19', '2018-09-11', 1.00000000000000),
('VSG19', '2018-09-29', 1.00000000000000),
('VSG19', '2018-10-16', 1.00000000000000),
('VSG19', '2018-10-25', 1.00000000000000),
('VSG19', '2018-11-02', 1.00000000000000);
--===============================================================
SELECT
*,
days_since_last_sale = ISNULL(DATEDIFF(DAY, MAX(td.date) OVER (ORDER BY td.date ROWS BETWEEN UNBOUNDED PRECEDING AND 1 PRECEDING), td.date), 0),
days_from_first_sale = ISNULL(DATEDIFF(DAY, MIN(td.date) OVER (ORDER BY td.date), td.date), 0)
FROM
#TestData td;
add a comment |
Your Answer
StackExchange.ifUsing("editor", function () {
StackExchange.using("externalEditor", function () {
StackExchange.using("snippets", function () {
StackExchange.snippets.init();
});
});
}, "code-snippets");
StackExchange.ready(function() {
var channelOptions = {
tags: "".split(" "),
id: "1"
};
initTagRenderer("".split(" "), "".split(" "), channelOptions);
StackExchange.using("externalEditor", function() {
// Have to fire editor after snippets, if snippets enabled
if (StackExchange.settings.snippets.snippetsEnabled) {
StackExchange.using("snippets", function() {
createEditor();
});
}
else {
createEditor();
}
});
function createEditor() {
StackExchange.prepareEditor({
heartbeatType: 'answer',
convertImagesToLinks: true,
noModals: true,
showLowRepImageUploadWarning: true,
reputationToPostImages: 10,
bindNavPrevention: true,
postfix: "",
imageUploader: {
brandingHtml: "Powered by u003ca class="icon-imgur-white" href="https://imgur.com/"u003eu003c/au003e",
contentPolicyHtml: "User contributions licensed under u003ca href="https://creativecommons.org/licenses/by-sa/3.0/"u003ecc by-sa 3.0 with attribution requiredu003c/au003e u003ca href="https://stackoverflow.com/legal/content-policy"u003e(content policy)u003c/au003e",
allowUrls: true
},
onDemand: true,
discardSelector: ".discard-answer"
,immediatelyShowMarkdownHelp:true
});
}
});
Sign up or log in
StackExchange.ready(function () {
StackExchange.helpers.onClickDraftSave('#login-link');
});
Sign up using Google
Sign up using Facebook
Sign up using Email and Password
Post as a guest
Required, but never shown
StackExchange.ready(
function () {
StackExchange.openid.initPostLogin('.new-post-login', 'https%3a%2f%2fstackoverflow.com%2fquestions%2f53252561%2ftsql-calculate-linear-weighted-moving-average-on-a-discrete-time-series%23new-answer', 'question_page');
}
);
Post as a guest
Required, but never shown
1 Answer
1
active
oldest
votes
1 Answer
1
active
oldest
votes
active
oldest
votes
active
oldest
votes
up vote
0
down vote
I'm not overly familiar with that particular calculation but from what I just read, you should be able to use "window frames" to calculate the "rolling information need to assign the weights to past sales.
Without seeing the actual formula you're applying, I can't say for sure weather or not it will work or not.
The following is just an example off the top of my head...
IF OBJECT_ID('tempdb..#TestData', 'U') IS NOT NULL
DROP TABLE #TestData;
CREATE TABLE #TestData (
product_id CHAR(5) NOT NULL,
[date] DATE NOT NULL,
sales_per_day DECIMAL(19,14) NOT NULL
);
INSERT #TestData (product_id, date, sales_per_day) VALUES
('VSG19', '2018-05-19', 1.00000000000000),
('VSG19', '2018-05-23', 1.00000000000000),
('VSG19', '2018-05-24', 2.00000000000000),
('VSG19', '2018-06-25', 1.00000000000000),
('VSG19', '2018-07-26', 1.00000000000000),
('VSG19', '2018-07-28', 1.00000000000000),
('VSG19', '2018-08-01', 1.00000000000000),
('VSG19', '2018-08-11', 1.00000000000000),
('VSG19', '2018-08-29', 1.00000000000000),
('VSG19', '2018-09-11', 1.00000000000000),
('VSG19', '2018-09-29', 1.00000000000000),
('VSG19', '2018-10-16', 1.00000000000000),
('VSG19', '2018-10-25', 1.00000000000000),
('VSG19', '2018-11-02', 1.00000000000000);
--===============================================================
SELECT
*,
days_since_last_sale = ISNULL(DATEDIFF(DAY, MAX(td.date) OVER (ORDER BY td.date ROWS BETWEEN UNBOUNDED PRECEDING AND 1 PRECEDING), td.date), 0),
days_from_first_sale = ISNULL(DATEDIFF(DAY, MIN(td.date) OVER (ORDER BY td.date), td.date), 0)
FROM
#TestData td;
add a comment |
up vote
0
down vote
I'm not overly familiar with that particular calculation but from what I just read, you should be able to use "window frames" to calculate the "rolling information need to assign the weights to past sales.
Without seeing the actual formula you're applying, I can't say for sure weather or not it will work or not.
The following is just an example off the top of my head...
IF OBJECT_ID('tempdb..#TestData', 'U') IS NOT NULL
DROP TABLE #TestData;
CREATE TABLE #TestData (
product_id CHAR(5) NOT NULL,
[date] DATE NOT NULL,
sales_per_day DECIMAL(19,14) NOT NULL
);
INSERT #TestData (product_id, date, sales_per_day) VALUES
('VSG19', '2018-05-19', 1.00000000000000),
('VSG19', '2018-05-23', 1.00000000000000),
('VSG19', '2018-05-24', 2.00000000000000),
('VSG19', '2018-06-25', 1.00000000000000),
('VSG19', '2018-07-26', 1.00000000000000),
('VSG19', '2018-07-28', 1.00000000000000),
('VSG19', '2018-08-01', 1.00000000000000),
('VSG19', '2018-08-11', 1.00000000000000),
('VSG19', '2018-08-29', 1.00000000000000),
('VSG19', '2018-09-11', 1.00000000000000),
('VSG19', '2018-09-29', 1.00000000000000),
('VSG19', '2018-10-16', 1.00000000000000),
('VSG19', '2018-10-25', 1.00000000000000),
('VSG19', '2018-11-02', 1.00000000000000);
--===============================================================
SELECT
*,
days_since_last_sale = ISNULL(DATEDIFF(DAY, MAX(td.date) OVER (ORDER BY td.date ROWS BETWEEN UNBOUNDED PRECEDING AND 1 PRECEDING), td.date), 0),
days_from_first_sale = ISNULL(DATEDIFF(DAY, MIN(td.date) OVER (ORDER BY td.date), td.date), 0)
FROM
#TestData td;
add a comment |
up vote
0
down vote
up vote
0
down vote
I'm not overly familiar with that particular calculation but from what I just read, you should be able to use "window frames" to calculate the "rolling information need to assign the weights to past sales.
Without seeing the actual formula you're applying, I can't say for sure weather or not it will work or not.
The following is just an example off the top of my head...
IF OBJECT_ID('tempdb..#TestData', 'U') IS NOT NULL
DROP TABLE #TestData;
CREATE TABLE #TestData (
product_id CHAR(5) NOT NULL,
[date] DATE NOT NULL,
sales_per_day DECIMAL(19,14) NOT NULL
);
INSERT #TestData (product_id, date, sales_per_day) VALUES
('VSG19', '2018-05-19', 1.00000000000000),
('VSG19', '2018-05-23', 1.00000000000000),
('VSG19', '2018-05-24', 2.00000000000000),
('VSG19', '2018-06-25', 1.00000000000000),
('VSG19', '2018-07-26', 1.00000000000000),
('VSG19', '2018-07-28', 1.00000000000000),
('VSG19', '2018-08-01', 1.00000000000000),
('VSG19', '2018-08-11', 1.00000000000000),
('VSG19', '2018-08-29', 1.00000000000000),
('VSG19', '2018-09-11', 1.00000000000000),
('VSG19', '2018-09-29', 1.00000000000000),
('VSG19', '2018-10-16', 1.00000000000000),
('VSG19', '2018-10-25', 1.00000000000000),
('VSG19', '2018-11-02', 1.00000000000000);
--===============================================================
SELECT
*,
days_since_last_sale = ISNULL(DATEDIFF(DAY, MAX(td.date) OVER (ORDER BY td.date ROWS BETWEEN UNBOUNDED PRECEDING AND 1 PRECEDING), td.date), 0),
days_from_first_sale = ISNULL(DATEDIFF(DAY, MIN(td.date) OVER (ORDER BY td.date), td.date), 0)
FROM
#TestData td;
I'm not overly familiar with that particular calculation but from what I just read, you should be able to use "window frames" to calculate the "rolling information need to assign the weights to past sales.
Without seeing the actual formula you're applying, I can't say for sure weather or not it will work or not.
The following is just an example off the top of my head...
IF OBJECT_ID('tempdb..#TestData', 'U') IS NOT NULL
DROP TABLE #TestData;
CREATE TABLE #TestData (
product_id CHAR(5) NOT NULL,
[date] DATE NOT NULL,
sales_per_day DECIMAL(19,14) NOT NULL
);
INSERT #TestData (product_id, date, sales_per_day) VALUES
('VSG19', '2018-05-19', 1.00000000000000),
('VSG19', '2018-05-23', 1.00000000000000),
('VSG19', '2018-05-24', 2.00000000000000),
('VSG19', '2018-06-25', 1.00000000000000),
('VSG19', '2018-07-26', 1.00000000000000),
('VSG19', '2018-07-28', 1.00000000000000),
('VSG19', '2018-08-01', 1.00000000000000),
('VSG19', '2018-08-11', 1.00000000000000),
('VSG19', '2018-08-29', 1.00000000000000),
('VSG19', '2018-09-11', 1.00000000000000),
('VSG19', '2018-09-29', 1.00000000000000),
('VSG19', '2018-10-16', 1.00000000000000),
('VSG19', '2018-10-25', 1.00000000000000),
('VSG19', '2018-11-02', 1.00000000000000);
--===============================================================
SELECT
*,
days_since_last_sale = ISNULL(DATEDIFF(DAY, MAX(td.date) OVER (ORDER BY td.date ROWS BETWEEN UNBOUNDED PRECEDING AND 1 PRECEDING), td.date), 0),
days_from_first_sale = ISNULL(DATEDIFF(DAY, MIN(td.date) OVER (ORDER BY td.date), td.date), 0)
FROM
#TestData td;
answered Nov 12 at 2:54


Jason A. Long
3,7151412
3,7151412
add a comment |
add a comment |
Thanks for contributing an answer to Stack Overflow!
- Please be sure to answer the question. Provide details and share your research!
But avoid …
- Asking for help, clarification, or responding to other answers.
- Making statements based on opinion; back them up with references or personal experience.
To learn more, see our tips on writing great answers.
Some of your past answers have not been well-received, and you're in danger of being blocked from answering.
Please pay close attention to the following guidance:
- Please be sure to answer the question. Provide details and share your research!
But avoid …
- Asking for help, clarification, or responding to other answers.
- Making statements based on opinion; back them up with references or personal experience.
To learn more, see our tips on writing great answers.
Sign up or log in
StackExchange.ready(function () {
StackExchange.helpers.onClickDraftSave('#login-link');
});
Sign up using Google
Sign up using Facebook
Sign up using Email and Password
Post as a guest
Required, but never shown
StackExchange.ready(
function () {
StackExchange.openid.initPostLogin('.new-post-login', 'https%3a%2f%2fstackoverflow.com%2fquestions%2f53252561%2ftsql-calculate-linear-weighted-moving-average-on-a-discrete-time-series%23new-answer', 'question_page');
}
);
Post as a guest
Required, but never shown
Sign up or log in
StackExchange.ready(function () {
StackExchange.helpers.onClickDraftSave('#login-link');
});
Sign up using Google
Sign up using Facebook
Sign up using Email and Password
Post as a guest
Required, but never shown
Sign up or log in
StackExchange.ready(function () {
StackExchange.helpers.onClickDraftSave('#login-link');
});
Sign up using Google
Sign up using Facebook
Sign up using Email and Password
Post as a guest
Required, but never shown
Sign up or log in
StackExchange.ready(function () {
StackExchange.helpers.onClickDraftSave('#login-link');
});
Sign up using Google
Sign up using Facebook
Sign up using Email and Password
Sign up using Google
Sign up using Facebook
Sign up using Email and Password
Post as a guest
Required, but never shown
Required, but never shown
Required, but never shown
Required, but never shown
Required, but never shown
Required, but never shown
Required, but never shown
Required, but never shown
Required, but never shown
aYDfgW81rWNL1e,eRQ xm76Fc4IlEOh1t 16QnYhr XtNX4aD2,r3h5GB54lPb1,nX
3
What's wrong with a calendar table? I would suggest that that is the normal (and elegant?) way to do so.
– Larnu
Nov 11 at 19:55
@Larnu is correct - that is the correct and elegant solution.
– Dale Burrell
Nov 11 at 20:18